Revisiting CycleGAN for semi-supervised segmentation - arXiv:1908.11569
Contents
Revisiting CycleGAN for semi-supervised segmentation - arXiv:1908.11569#
Information
Title: Revisiting CycleGAN for semi-supervised segmentation, arXiv:1908.11569
Reference
Review By: 김소연
Edited by: Taeyup Song
Last updated on Jan. 5, 2022
Background#
Semi-supervised Learning
적은 수의 Labeled dataset \(\mathcal{L}=\{(x_i,y_i)\}_{i=0}^{n}\)와 많은 수의 unlabeled dataset \(\mathcal{U}=\{x_i'\}_{i=0}^m\)를 이용하여 특정 task를 수행하는 neural network를 학습
Labeled dataset에 대해서는 supervised learning으로 학습 가능, unlabeled dataset으로 regularization.
GAN (Generative Adversarial Network)
Semi-supervised learning 및 unsupervised domain adaptation에서 많이 적용됨.
CycleGan
반복적으로 입/출력되는 형태로 구성된 GAN
domain 간의 image style transfer를 위해 사용됨 → 학습 시 consistency loss를 적용하여 image pair restriction 없이 학습 가능 (domain간의 pair 없이도 학습 가능) → Semi-supervised segmantation task는 labeled set과 unlabeled set의 domain이 다르지 않기 때문에 CycleGAN이 적용되지 않았음.
Contribution#
CycleGAN의 unpair domain adaptation ability를 이용하여 “unlabeled real image”에서 GT mask와 다시 image로 mapping하는 방법을 학습.
Unlabeld dataset에 대해 적용 가능한 unsupervised regularization loss 제안
기존 GAN 적용 semi-supervised semantic segmentation과 달리 CycleGAN을 이용하여 unlabed image와 GT mask간의 cycle consistent mapping을 수행.
Proposed Method#
1. CycleGAN for semi-supervised segmentation#
두 개의 conditional generator와 discriminator로 구성됨.
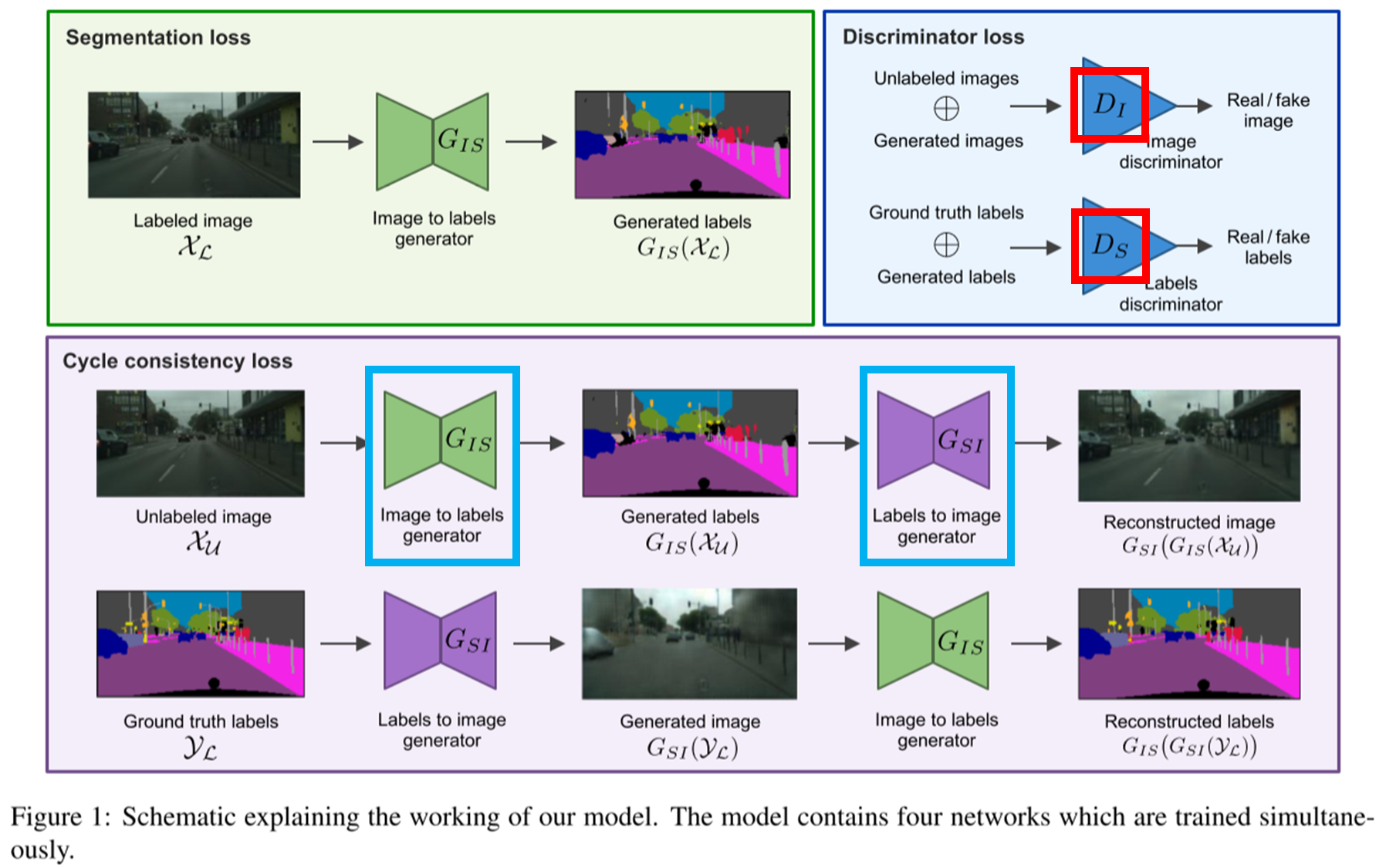
Fig. 127 Schematic explaining the working of proposed model (source: arXiv:1908.11569)#
2. Loss functions#
(1) For labeled dataset \(\mathcal{X}_{\mathcal{L}}\)
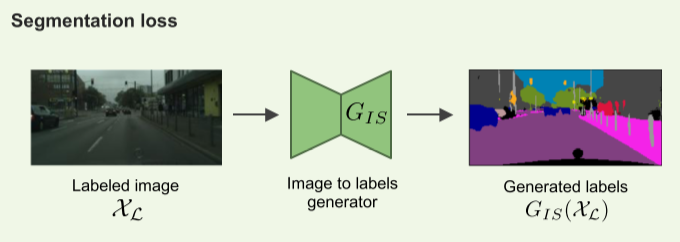
Fig. 128 Segmentation loss (source: arXiv:1908.11569)#
labeled data에 대해서는 pixel level의 classification을 위해 Cross-entropy Loss를 적용
\[L_{\text{gen}}^S(G_{IS})=\mathbb{E}_{x,y\backsim \mathcal{X}_{\mathcal{L}} \mathcal{Y}_{\mathcal{L}}}\left[\mathcal{H}(y,G_{IS}(x)) \right]\]ㅏㅏ여기서 \(\mathcal{H}\)는 pixel \(j\)가 \(k\) class에 속할 확률을 나타낸다.
labeled image와 이에 해당하는 GT로 생성된 image간에 loss는 \(L2\) norm을 적용
\[L_{\text{gen}}^I(G_{SI})=\mathbb{E}_{x,y\backsim \mathcal{X}_{\mathcal{L}} \mathcal{Y}_{\mathcal{L}}}\left[ ||G_{SI}(y)-x||^2_2\right]\]
(2) Adversarial loss for unlabeled dataset
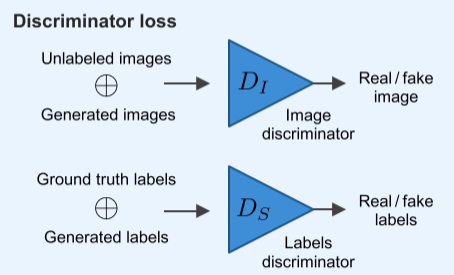
Fig. 129 Adversarial loss (source: arXiv:1908.11569)#
Generator G와 Discriminator D의 compete
unlabeld image와 GT로 부터 생성된 image를 구분하기 위한 discriminator \(D_I\)을 위한 adversarial loss는 학습의 용의성을 위해 square loss 적용
\[L_{\text{disc}}^I(G_{SI},D_I)=\mathbb{E}_{x\backsim \mathcal{X}_{\mathcal{U}}}\left[ (D_I(y)'-1)^2\right]+\mathbb{E}_{y\backsim \mathcal{Y}_{\mathcal{L}}}\left[ (D_I(G_{SI}(y)))^2\right]\]여기서 \(D_I(x)\)는 image \(x\)가 진짜 image일 확률을 나타냄.
GT labels와 unlabeled image로 부터 생성된 labels를 구분하기 위한 discriminator \(D_S\)를 위한 adversarial loss 역시 square loss 적용
\[L_{\text{disc}}^S(G_{IS},D_S)=\mathbb{E}_{y\backsim \mathcal{Y}_{\mathcal{L}}}\left[ (D_S(y)-1)^2\right]+\mathbb{E}_{x\backsim \mathcal{X}_{\mathcal{U}}}\left[ (D_S(G_{IS}(x')))^2\right]\]여기서 \(D_S(y)\)는 label \(y\) 가 진짜 mask일 확률을 나타냄.
(3) Cycle consistency loss for unlabeld dataset
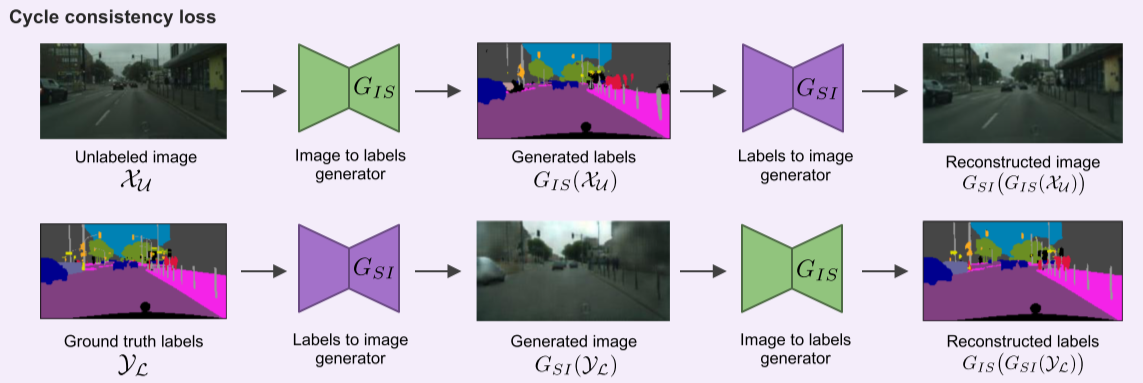
Fig. 130 Cycle consistency loss (source: arXiv:1908.11569)#
Unlabeled data \(\mathcal{X}_{\mathcal{U}}\)를 generator \(G_{IS}\)로 label을 생성하고, 다시 generator \(G_{SI}\)를 이용하여 복원한 cycle의 output에 대해 L1 norm 적용 (최종 image를 sharper 하게 만들기 위함)
Ground truth로부터 Generator \(G_{SI}\)로 영상을 생성하고, 다시 generator \(G_{IS}\)로 labels를 복원하는 cycle의 경우 분류 문제에 해당하므로 cross-entropy 적용
3. Implementation Details#
Experimental Result#
1. Setting#
Dataset
PASCAL VOC 2012 - object / 200x200 pixel로 resize하고 feed 함
Cityscapes - scene / 128x256 pixel로 resize
ACDC - medical image
Fully supervised learning으로 학습하여 upper bound performance 구함
Labeled을 10, 20, 30, 50%를 사용하여 partial baseline 생성
이전 SOTA와 비교: Adversarial learning for semi-supervised semantic segmentation
2. Result & discuss#
20%의 label을 이용한 결과에서 기존 SOTA 방법의 경우 Partial baseline 대비 성능이 저하되지만, 제안된 방법의 경우 더 높은 성능을 나타냄
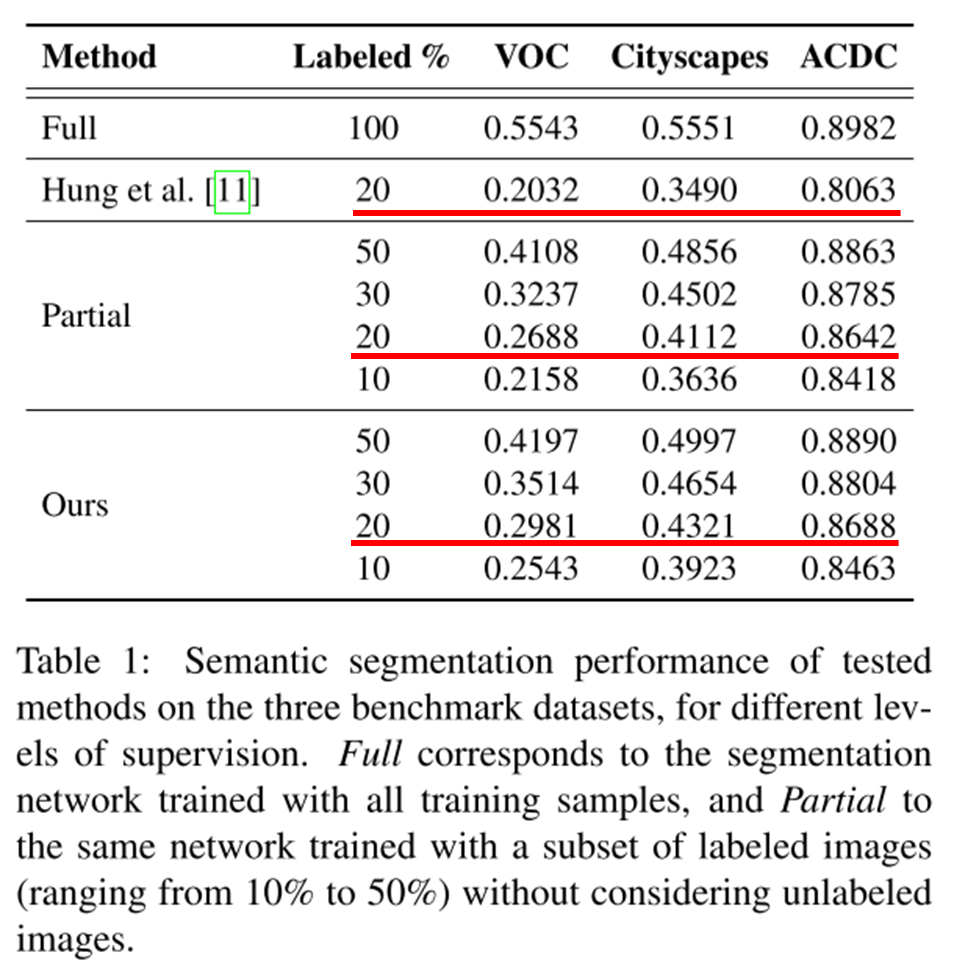
full supervision을 적용한 baseline 대비 정확도는 낮아도, global semantic과 디테일은 잘 capture한다고 주장
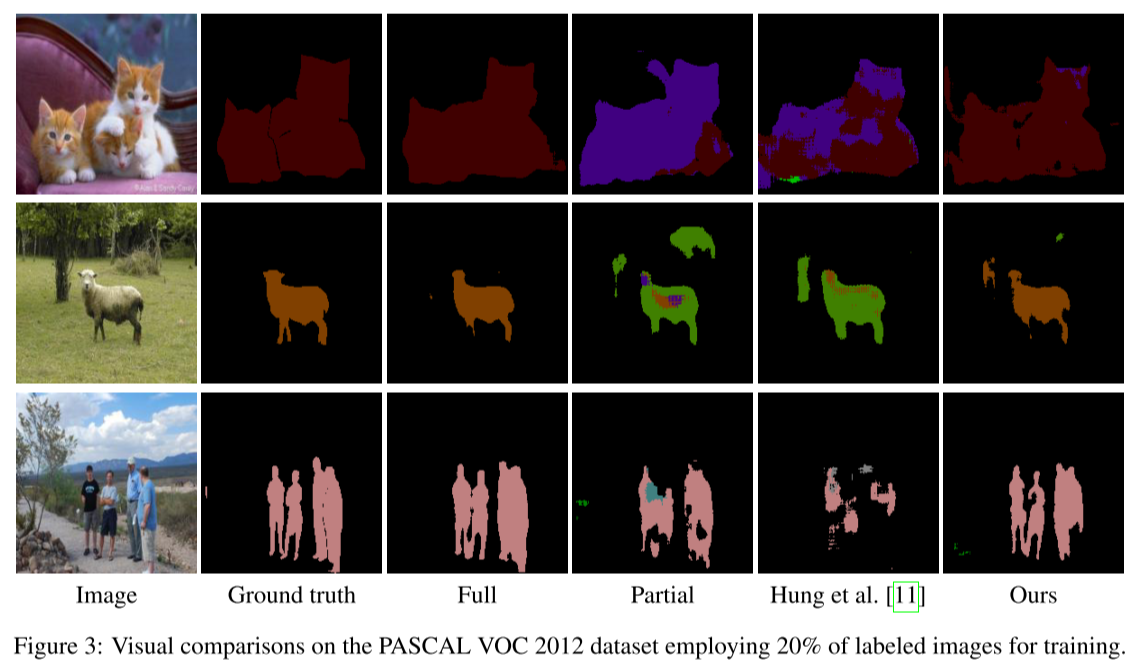
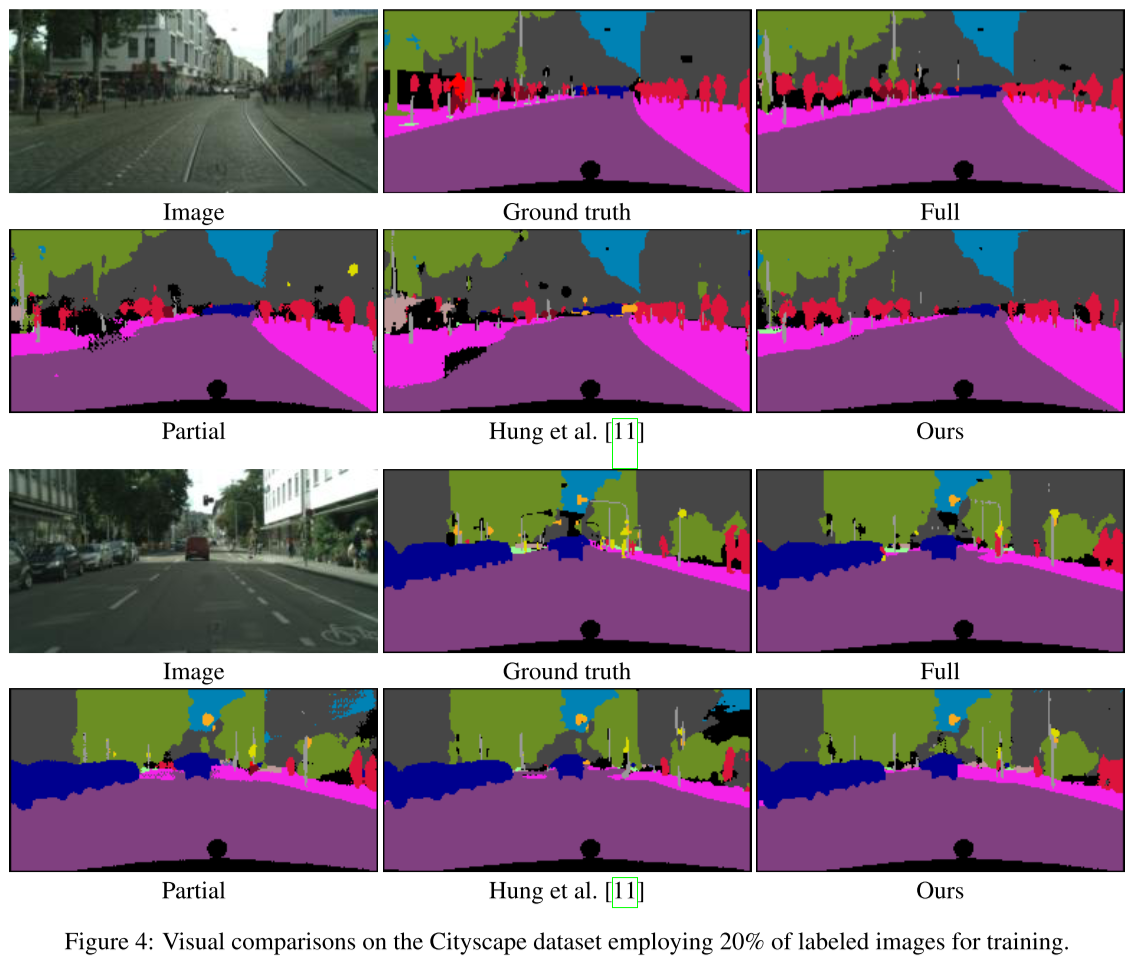
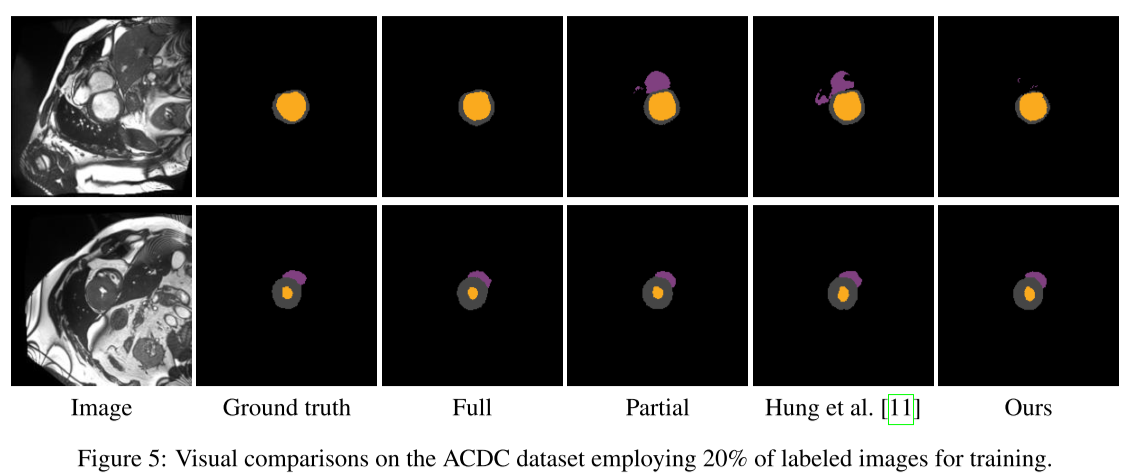
Cycle loss의 경우 consistency loss on segmentation mask가 성능에 직접적인 영향을 줌. 즉 mask로 image를 생성하고 다시 mask를 생성했을 때, 기존과 동일한지 여부가 중요함
Discriminator loss의 경우 image discriminator가 더 성능에 큰 영향을 미침. 즉 생성된 이미지인지, real unlabeled 이미지인지 구분하는 판단이 더 중요함.
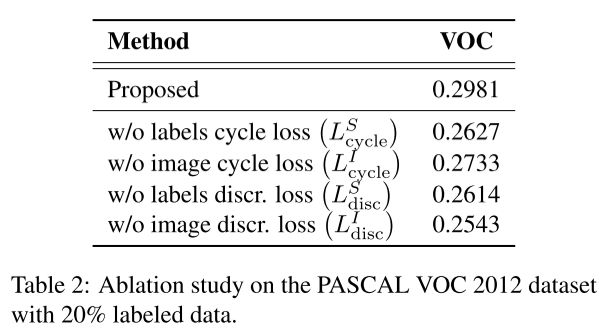