Segformer - NeurIPS 2021
Contents
Segformer - NeurIPS 2021#
Information
Title: SegFormer: Simple and Efficient Design for Semantic Segmentation with Transformers, NeurIPS 2021
Reference
Review By: Jongsu Choi
Edited by: Taeyup Song
Last updated on July. 10, 2022
Introduction#
이미 자연어처리 분야에서 효과를 입증받은 Transformer 구조는 ViT(Vision Transformer) 와 같이 image classification task를 시작으로 semantic segmentation과 같은 다양한 computer vision task에 적용되고 있다.
SETR과 같이 ViT를 backbone으로 사용하는 연구들은 다음과 같은 대표적인 문제가 있다.
Single-scale low-resolution feature-map을 사용함.
높은 computational cost를 가짐
encoder 디자인에 치중하여 decoder의 기여도를 평가 절하.
위와 같은 문제를 해결하기 위해 본 연구에서는 다음과 같은 방법을 제안하였다.
(1) No Positional Encoding
Positional encoding은 학습에 사용된 이미지와 다른 해상도를 가진 이미지를 inference에서 사용시 성능저하를 유발한다.
(2) Hierarchical Transformer
Encoding된 feature들 중 고해상도(high resolution)의 fine feature와 저해상도(low resolution)의 coarse feature를 모두 학습이 가능하게 하였다.
(3) Light Weight MLP Decoder
Transformer의 low layer에서 얻어지는 local Information과 high layer에서 얻어지는 global information을 aggregating 하는 구조로 powerful한 성능을 보인다.
SETR 대비 적은 parameter를 사용하면서도 ADE20K DB에서 SOTA 성능을 타나냄.
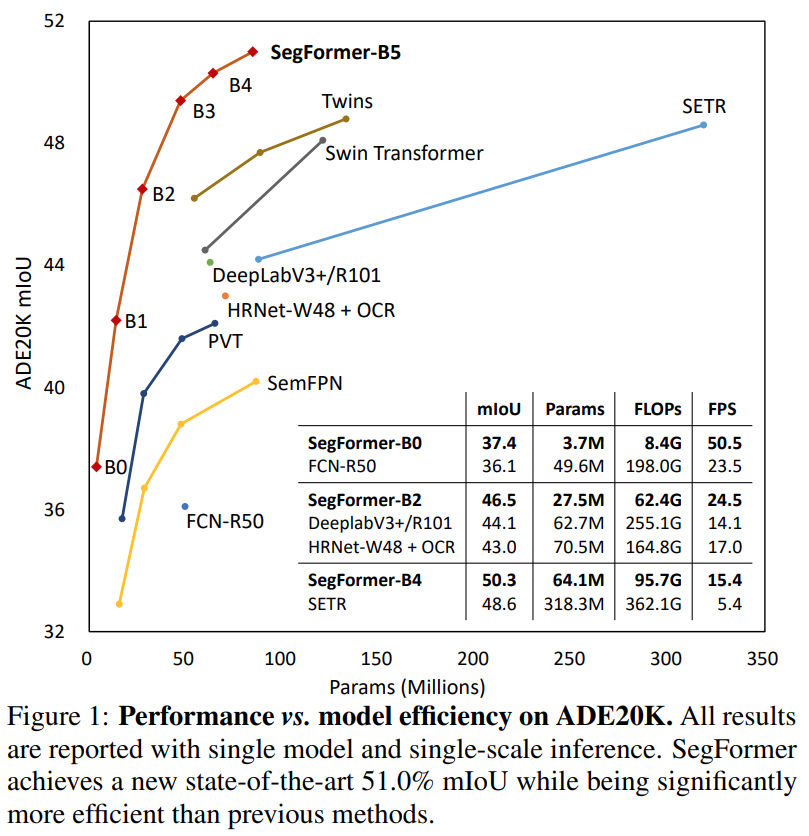
Fig. 36 Performance vs. model efficiency (source: arXiv:2105.15203)#
Proposed Method#
1. SegFormer vs SETR#
Training Data : ImageNet 1K vs 22K
Encoder : Hierarchical architecture vs Single resolution feature map
Patch Size: 4x4 vs 16x16
Positional Information : No PE(Mix FNN) vs PE(Positional Encoding)
Decoder : “Negligible” computational overhead decoder
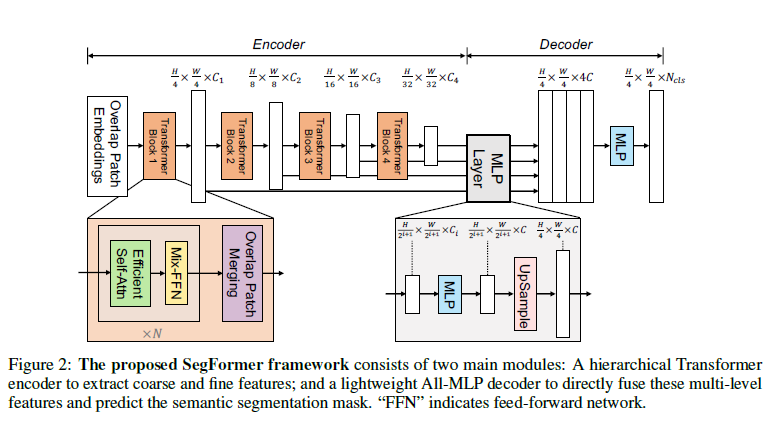
Fig. 37 The proposed SegFormer framework (source: arXiv:2105.15203)#
2개의 Main 모듈로 구성 : (1) Hierarchical Transformer Encoder & (2) Light weight MLP decoder
2**. Hierarchical Transformer Encoder**#
(1) Hierarchical Feature Representation
Overlapped patch merging 방법을 활용해 CNN 구조에서와 같이 multi-level feature를 뽑는 것이 목표 (기존 ViT : Single Resolution Feature map)
High-resolution feature에서는 coarse 한 정보, low resolution feature에서는 fine한 정보를 추출하고, 이를 디코더에 융합하여 segmentation 성능을 높인다.
본 논문에서는 4-level feature를 추출하며, 각 \(i\)번째 level의 feature는 \(\frac{H}{2^{i+1}}\times \frac{W}{2^{i+1}}\times C_i\) resolution을 갖는다.
(2) Overlapped Patch Merging
ViT는 sequentialization을 위해 1개의 \([N \times N \times 3]\) (\(N=16\)) 패치를 \([1 \times 1 \times C]\) 형태로 flatten하여 표현하는데, 각 patch가 overlap 되지 않기 때문에 local continuity를 보존하기 어려운 문제가 있다.
본 논문에서는 local continuity를 보존하기 위해 stride/padding 개념과 유사하게 overlap된 patch를 추출하고 merge한다.
이를 위해 parameter \(K\) (patch size), \(S\)(stride), \(P\)(padding size)를 정의하였고, 논문에서는 \(K=7\), \(S=4\), \(P=3\)과 \(K=3\), \(S=2\), \(P=1\)으로 설정한다.
(3) Efficient Self-Attention
\(N \times C=H \times W \times C\) dimension의 query \(Q\), key \(K\), value \(V\)가 주어질 때 Multi-head self attention은 다음과 같이 나타낼 수 있다.
\[ \text{Attention}(Q,K,V)=\text{Softmax}\left( \frac{QK^T}{\sqrt{d_{head}}}\right)V \]Attention은 \(O(N^2)\)의 computation complexity를 가지므로, main bottleneck으로 작용하고, VIT의 16x16 patch에 비해 작은 4x4 patch를 적용하기 때문에 연산량이 더 커진다.
Reduction ratio \(R\)을 사용하여 sequence length를 줄이는 방식으로 개선한다. [8]
\[\begin{split} \begin{aligned} \hat{K}&=\text{Reshape}\left(\frac{N}{R},C\cdot R\right)(K) \\ K&=\text{Linear}\left(C\cdot R,C\right)(\hat{K}) \end{aligned} \end{split}\]여기서 \(K\)는 length를 줄이고자 하는 sequence이고, \(\text{Reshape}(B, C)(A)\)는 \(A\)를 \((B \times C)\)으로 shape을 변환하는 연산이며, \(\text{Linear}(C_{in}, C_{out})(\cdot)\)은 \(C_{in}\) dimension의 tensor를 입력받아 \(C_{out}\) dimension tensor의 출력을 내는 연산이다.
위 연산에 의해 새로운 sequence \(K\)는 \((\frac{N}{R}\times C)\) dimension을 갖으며, self-attention의 연산량을 \(O(N^2/R)\)으로 개선할 수 있다.
(질문 R^2가 아닌 이유)본 논문에서는 stage 1부터 4까지 실험을 통해 얻은 reduction ratio \(R=[64, 16, 4, 1]\)을 적용한다.
(4) Mix FFN
기존에는 위치 정보를 위해 PE(positional encoding)를 사용하였는데 이는 학습에서 사용된 이미지와 다른 해상도의 테스트 이미지가 사용되었을 때 별도의 interpolation이 요구됨으로 성능 저하를 유발한다.
본 논문에서는 semantic segmentation task에서는 PE가 필요 없다고 주장하며, 이를 대신하여 3x3 covolution을 FFN에 적용하여 PE를 대체하는 Mix-FFN구조를 제안함. (conv. 연산시 zero padding을 적용하면 leak location information을 대체할 수 있다고 주장함. [69])
\[ \mathbf{x}_{out}=\text{MLP}(\text{GELU}(\text{Conv}_{3\times3}(\text{MLP}(\mathbf{x}_{in}))))+\mathbf{x}_{in} \]Mix-FFN은 3 X 3 conv. layer를 통해 positional 정보를 추출하고 이것을 더해주는 것으로도 좋은 성능을 나타냄을 확인함.
3 Light weight All-MLP Decoder#
본 모델에서 제시한 hierarchical encoder가 기존 CNN대비 큰 ERF(Effective Receptive Field)를 가지므러, 단순한MLP구조로도 decoder를 구성하여 연산 효율을 높인다.
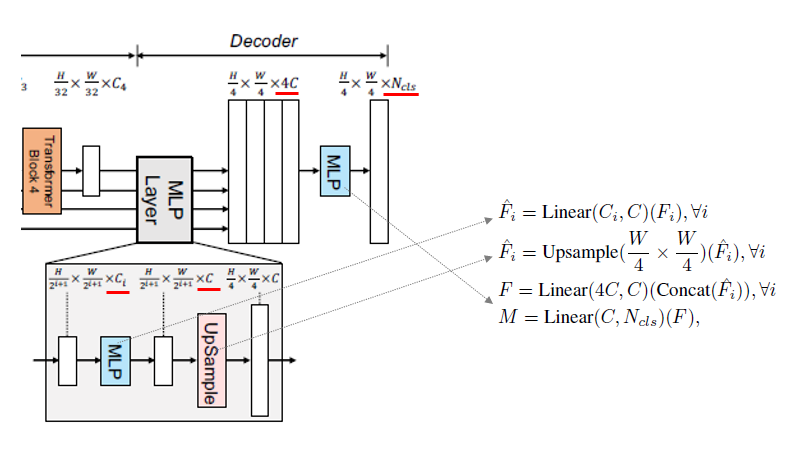
Fig. 38 Decoder structure (source: arXiv:2105.15203)#
4개의 Step으로 구성 :
(1) Hierarchical Encoder에서 나온 서로 다른 채널을 가진 4개의 Feature Map을 각각 MLP layer를 거처 C로 통일된 채널로 만들어 준다.
(2) Up-Sampling 하여 \([H/4 \times W/4 \times C]\)로 만들어 준 후 Concatenate하여 4개의 Feature Map을 하나로 합쳐준다.
(3) Concatenate된 정보를 융합하기 위해 MLP를 거친다.
(4) 최종적으로 MLP를 거쳐 \(N\)(# of Classes)로 Feature Map 채널 변경.
ERF(Effective Receptive Field) Analysis
Semantic Segmentation에서 Context 정보를 Capture하기 위해 Receptive Field를 크게 하는 것이 큰 이슈이다.
본 연구는 통해 Transformer의 Non-Local Attention을 통해 Large Receptive Field를 확보하고, Decoder를 통해 Local and Non-Local Attention을 동시에 잡아 낼 수 있었다.
Fig. 39 Effective Receptive Field on Cityscapes (source: arXiv:2105.15203)#
결과 해석 (각 Stage는 Hierarchical Encoder Block)
(1) DeepLab V3+는 Segformer 대비 상대적으로 작은 ERF를 가진다.
(2) Segformer도 앞 Stage에서는 자연스럽게 Local Attention에 집중 되는데, 뒤로 갈 수록 Non-Local Attention을 효과적으로 뽑아내 Context Information을 잡아낸다. (3) Decoder Head를 거쳐 Non-local Attention과 더불어 Local Attention에 더 집중 하게 된다.
Experiment#
1. Implementation Details#
mmsegmentation 기반으로 구현
Augmentation:
Random cropping 512x512: ADE20K and COCO stuff 1024x1024: Cityscapes 640x640: ADE20K for B5 model
Random horizontal flipping (resize ratio: 0.5~2.0)
Optimization
Init. learning rate: 0.00006 (on ADE20K, Pascal Context), 0.01 (on Cityscapes)
polynomial learning rate schedule (factor 1.0)
Momentum = 0.9, weight decay =0
Encoder : imagenet 1K decoder random initialize
2. Result#
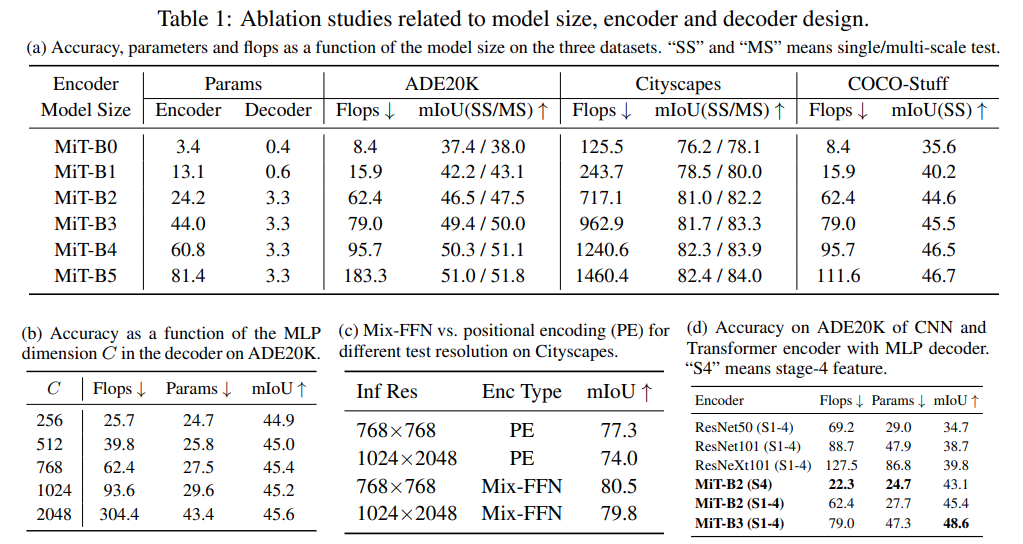
Transformer encoder의 layer가 커지면 연산량은 커지지만 성능이 향상됨을 확인. B0(Light Weight) ~ B5(Largest Weight but Best Performance) (Table 1. (a))
Decoder의 channel을 256에서 더 늘려도 큰 효과가 없음. 실험적으로 channel수가 768 일 때 가장 좋은 성능을 보여 B2~B5 model은 이 값을 사용함. (Table 1. (b))
PE(Positional Encoding) 유무에 따른 성능을 비교한 결과 이미지 resolution 변화에 대해서 inference에서 PE 대비 더 robust한 결과를 나타냄. ((Table 1. (c))
(d) MLP decoder가 더 큰 receptive field를 가지는 Transformer encoder에 적합함을 확인. CNN이 Transformer 대비 좁은 receptive field를 가지기 때문에 MLP decoder만으로 global한 추론을 하기에 부족함이 있다.
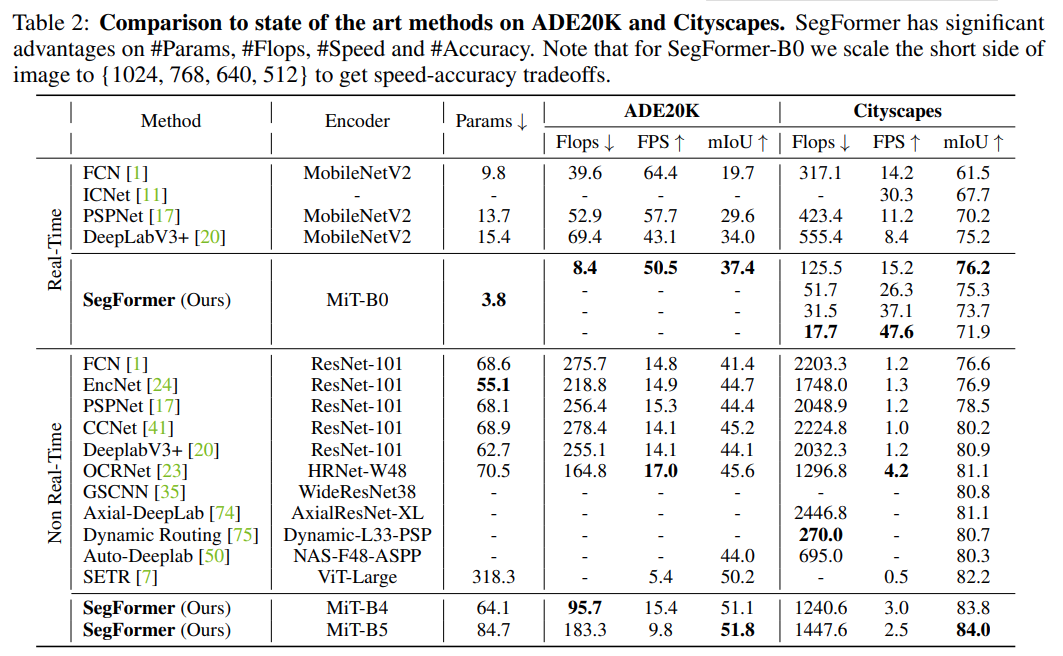
ADK20K dataset에서 SegFormer-B0는 3.8M parameter만 사용하고, 37.4%의 mIoU를 기록하여, real-time으로 동작하는 FCN, PSPNet, DeepLabV3+보다 적은 parameter를 사용하고도 높은 성능을 타나냄을 확인할 수 있다.
Non Real-Time으로 동작하는 기존 SOTA method인 AutoDeepLab, SETR와 비교하여 SegFormer-B4 model이 낮은 FLOPs를 가지면서도 높은 성능을 나타냄을 확인할 수 있다.
Conclusion#
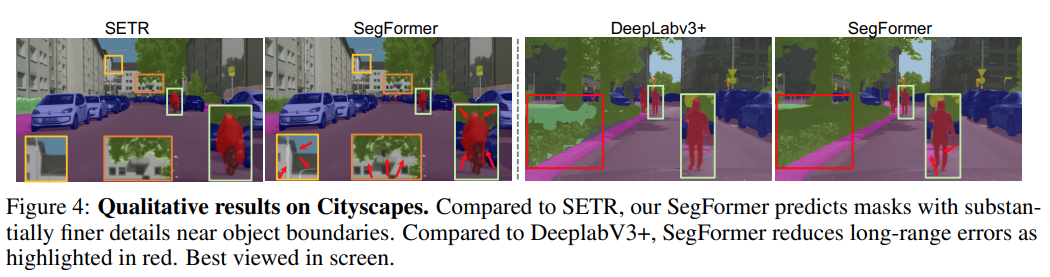
Simple, Clean, Powerful, SOTA, Strong Zero-shot robustness
One Limitation : 3.7M parameters (b0 기준)뿐이지만, 아주 작은 메모리를 가진 작은 device에서도 잘 작동하는지 확인이 필요한 한계가 존재한다.