DETR - ECCV 2020
Contents
DETR - ECCV 2020#
Information
Title: End-to-End Object Detection with Transformers, ECCV 2020
Reference
Review By: Taeyup Song
Edited by: Taeyup Song
Last updated on Jan. 30, 2022
Problem Statement#
Object detection task는 bounding box와 label을 예측하는 것인데, 최근의 연구 결과들은 anchor를 기반으로 bbox regression/classification을 수행하는 휴리스틱한 방법을 주로 사용한다.
결국 Target object의 bounding box의 aspect ratio와 같은 prior knowledge에 의존하고, 중복 검출을 제거하기 위해 NMS에 많은 의존을 하게 되어 occlusion이 발생한 object를 검출하기 어렵다. 에 되는 문제가 있음.
RNN을 적용한 detector들은 auto-regressive(자기추론) sequence model을 이용하기 때문에 loss function은 변하지않는 순열의 prediction한다. 다만 최신 기준으로 평가된 적이 없으며, 병렬처리가 어려운 단점이 있다.
Contribution#
Transformer를 이용한 end-to-end detection framework를 제안함.
Unique prediction을 갖는 detector로 기존 detector가 가지는 휴리스틱한 로직 없이 Faster R-CNN과 동등한 성능을 가짐.
Transformer로 간단하게 architecture 만들어져서 쉽게 확장 가능함.
→ Panoptic Segmentation Task ..
→ ViT, Deformable DETR, Swin transformer

Fig. 75 DETR directly predicts (in parallel) the final set of detections by combining a common CNN with a transformer architecture (source: arXiv:2005.12872)#
Proposed Method#
1. Overall Architecture#
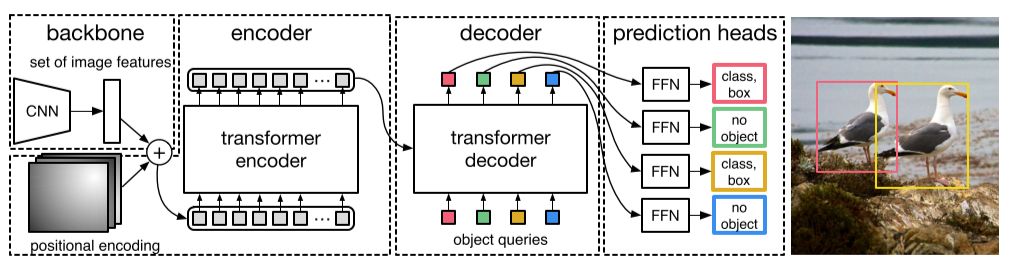
Fig. 76 DETR uses a conventional CNN backbone to learn a 2D representation of an input image. (source: arXiv:2005.12872)#
CNN backbone (ResNet)을 이용하여 feature를 추출한 후, transformer의 encoder-decoder를 통해 얻은 attention을 FFN(feed forward network)를 통해 고정된 수의 detection box와 label을 얻음
FFN은 perceptron으로 이루어진 neural network이며 ReLU activation을 적용한 3 layer network로 구성된다.
2. Transformer#
CNN을 이용하여 추출한 feature map에서 bounding box와 이에 따른 class 정보를 예측하기 위해 Transformer architecture를 이용한다. Transformer는 적은 inductive bias를 가지는 구조로 global context를 이해하는데 좋은 성능을 발휘한다고 알려져 있다.
Transformer의 encoder에서는 입력된 feature에 대한 self-attention을 이용하여 objects의 instance level의 정보를 추출한다.
Transformer의 decoder는 N개의 objects에 대한 queries를 input으로 받으며, 각 object의 localiztion information을 예측한다. 이 과정에서 parallel decoding을 이용하여 연산을 가속할 수 있다.
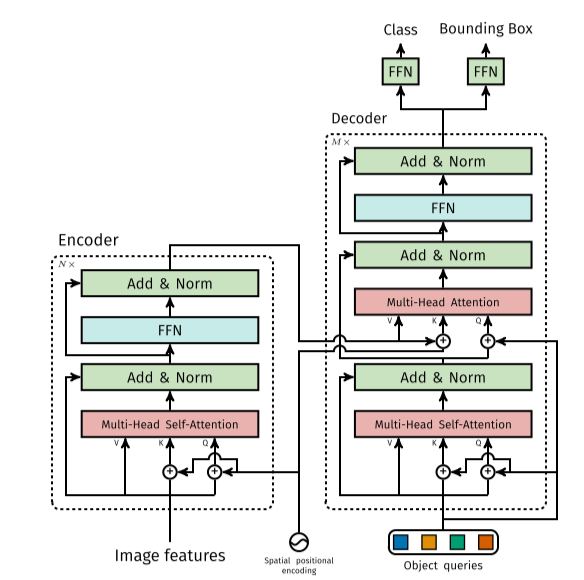
Fig. 77 Architecture of DETR’s Transformer. (source: arXiv:2005.12872)#
Encoder의 output은 각 개별 object instance 정보를 포함하고 있다. 그림 Fig. 78와 같이 붉은 점에 해당하는 self-attention을 보면 각 위치가 포함되는 개별객체에 attention되어 있음을 확인할 수 있다.
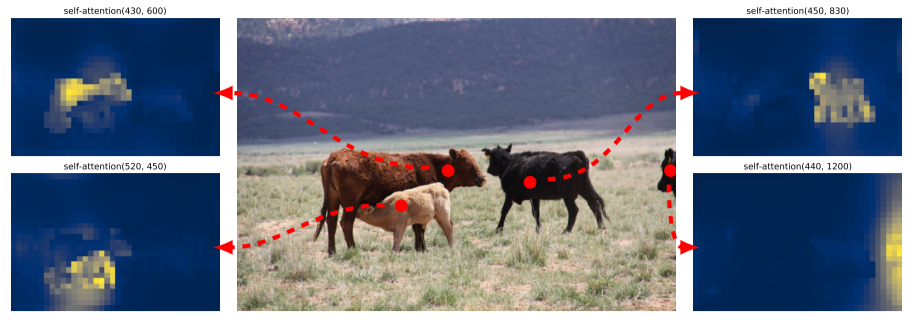
Fig. 78 Encoder self-attention for a set of reference points. The encoder is able to seperate indifidual instancese. (source: arXiv:2005.12872)#
Fig. 79와 같이 DETR는 중첩된 object도 잘 구분할 수 있다.
각 instance의 외각점 (다리, 귀, 코 등)에서 높은 attention을 가진다.
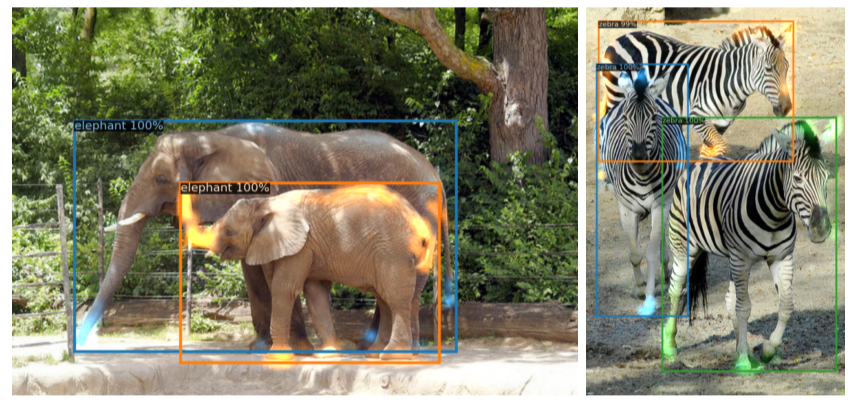
Fig. 79 Visualizing decoder attention for every predicted object (image from COCO val set). Predictions are made with DTRE-DC5 model. Attention scores are coded with differentn colors for different objects. Decoder typically attends to object extremities, such as legs and heads. Best viweed in color (source: arXiv:2005.12872)#
3. Bipartite Matching#
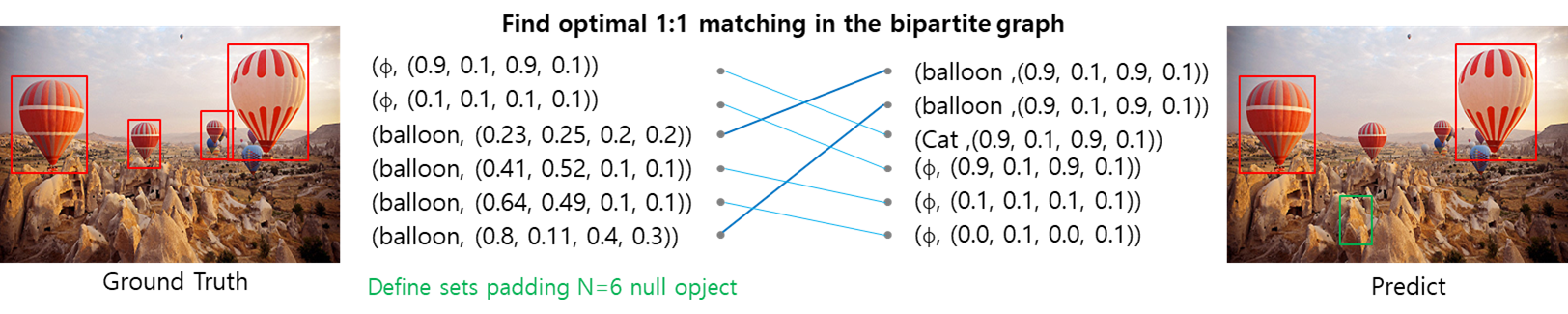
Fig. 80 Examples of bipartite matching (source: arXiv:2005.12872)#
Matching cost
을 최소화하는 최적의 permutation of element 을 찾아 1:1 matching을 수행한다. 이 과정은 Hungarian algorithm을 이용하여 효율적으로 계산할 수 있다.
Ground truth
는 개의 prediction set구성되어 있다. 만약 실제 영상 내 object가 보다 작은 경우 개의 set이 되도록 ∅(no object)가 추가된다. 클래스 label 와 bbox의 center coordinates 를 가지는 object 와 prediction 에 대해 matching loss 는 다음과 같이 구성된다.여기서
는 permutation of N elements이며, 는 class 에 속할 확률이다.
4. Loss Function#
DETR 학습을 위해 다음과 같이 Hungarian loss를 계산한다.
여기서
는 bipartite matching 과정을 통해 얻은 optimal assignment 이다.DETR는 anchor를 적용하지 않고 bounding box를 바로 prediction하기 때문에 오차가 커질 수 있고, 기존 detector가 bbox regression에 사용하는 L1 loss는 bbox가 클수록 오차가 커지는 문제점이 있다. 이러한 문제점을 보완하고자 L1 loss와 generalized IOU
를 이용하여 box prediction loss를 정의한다.
5. Panoptic Segmentation#
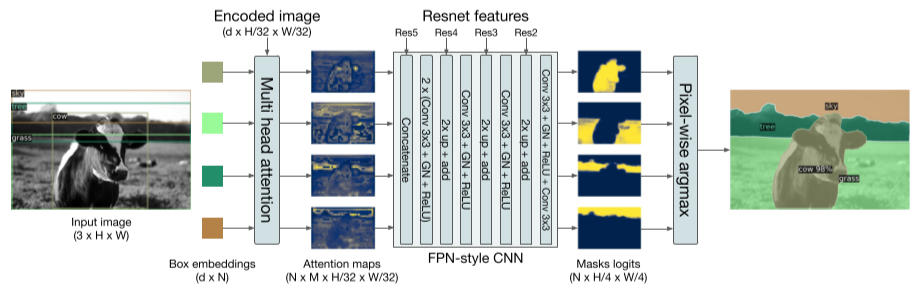
Fig. 81 Illustration of the panoptic head. A binary mask is generated in parallel for each detected object, then the masks are merged using pixel-wise argmax. (source: arXiv:2005.12872)#
DETR의 Transformer decoder output에 mask head를 추가하여 Panoptic Segmentation을 수행 가능하다.
Detection task와 같이 DETR를 이용하여 stuff, things class를 각각 학습하고, 각 prediction boxes에 대해 binary mask를 prediction한다.
Transformer decoder에서 stuff, thing에 대한 detection 결과가 도출되면, 이를 저장해둔 Transformer의 encoder output을 이용하여 attention을 계산한다. (그림 x의 multi-head attention block)
각 prediction box에 대해 구한 M개의 attention heatmap을 FPN과 유사한 CNN architecture를 이용하여 해상도가 개선된 masks logits을 도출한다. (최종적으로 prediction된 mask는 stride 4의 크기를 갖도록 설정됨.)
최종적으로 구한 Mask logits에 대해 pixel-wise argmax를 적용하여 최종 panotic segmentation 결과를 도출한다.
Mask head는 DETR와 함께 학습되거나, 분리되어 학습할 수 있다. 실험 결과 유사한 성능을 나타낸다고 한다. 각 mask는 독립적을 DICE/F-1 loss와 Focal loss를 적용하여 학습한다.
Experimental Result#
1. Object Detection#
Traning details - COCO 2017 detection dataset training 118k, vaildation 5k
Learning rate of transformer 1e-4
Learning rate of backbone: 1e-5
Weight decay: 1e-4
V100 x 16, 3 days for 300 epoch (batch size = 64) in case of basemodel
DC5: dilated C5 stage, adding a dilation to the last stace of the backbone to increase the feature resolution
Discuss
DETR DC5-R101의
와 같이 작은 object에 대해 성능이 저하됨을 확인할 수 있음
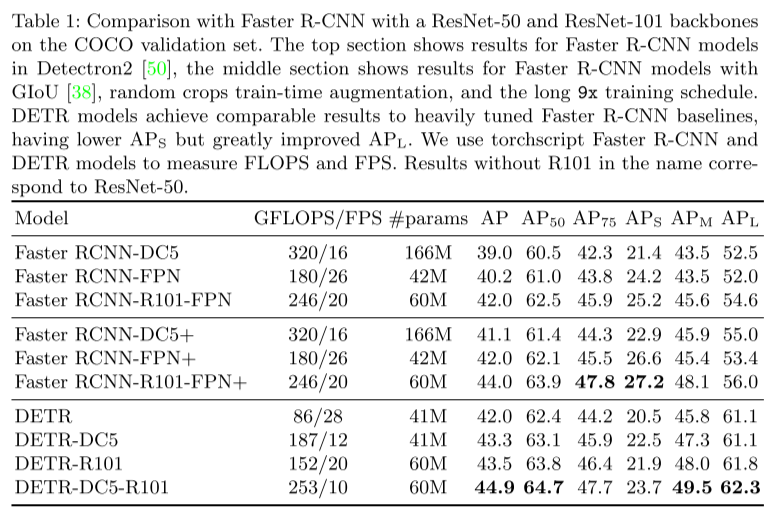
2. Panoptic Segmentation#
Traning details - COCO 2017 panotic dataset training (80categories에 53 stuff categories추가)
25 epoch in case of mask head
Discuss
Baseline인 PanopticFPN과 비교하여 높은 성능을 나타내며, 특히 stuff class에 대한 결과인
에서 baseline 대비 좋은 성능을 보임Encoder의 attention을 이용하여 global reasoning을 효과적이며, segmentation task에도 DETR 구조가 효용이 있음을 나타냄.
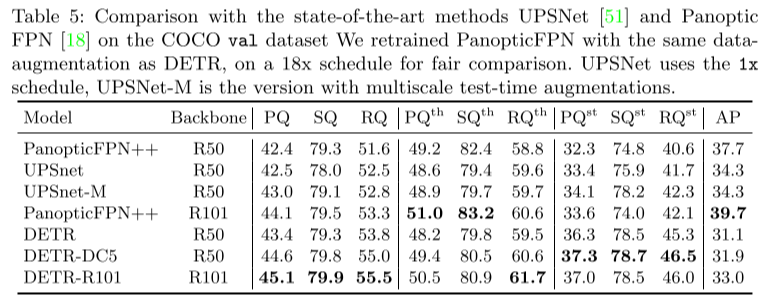
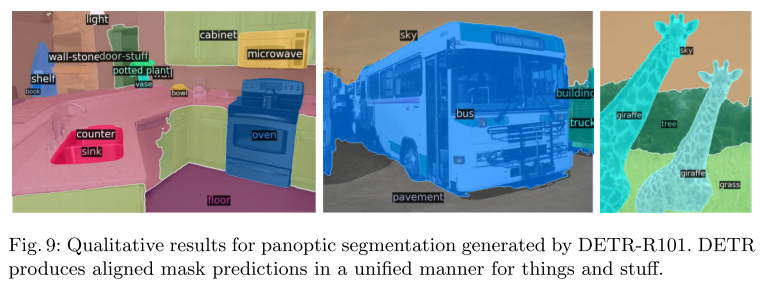