IAL - IJCV 2020
Contents
IAL - IJCV 2020#
Information
Title: Weakly-Supervised Semantic Segmentation by Iterative Affinity Learning, IJCV 2020
Reference
Paper: https://arxiv.org/abs/2002.08098 (International Journal of Computer Vision (IJCV), 2020)
Code: https://github.com/WangXiang10/IAL (empty repo..)
Review By: Taeyup Song
Edited by: Taeyup Song
Last updated on Jan. 5, 2022
Reference#
Paper: https://arxiv.org/abs/2002.08098 (International Journal of Computer Vision (IJCV), 2020)
Code: https://github.com/WangXiang10/IAL (empty repo..)
Contribution#
각 pixel의 Label probability를 학습하기 위한 Unary segmentation network와 이를 refine하기 위한 pairwise affinity network로 구성된 architecture 제안
정확한 annotation 없이 신뢰성 높은 pixel affinity를 학습하기 위한 mining confident regions 기법과, 제안한 framework과 equivalent한 energy function을 local min.에 수렴시키기 위해 iteratively하게 학습을 수행하는 방법을 제안.
PASCAL VOC 2021 및 COCO dataset에서 좋은 성능을 나타냄을 확인.
Proposed Method#
1. Architecture#
각 pixel \(x\)의 label probability를 구하기 위한 Unary network \(F=f(x,W_f)\)과 pixel affinity를 학습하기 위한 pairwise network \(G=f(x,W_g), G \in \mathcal{R}^{N \times N}\) 로 구성됨.
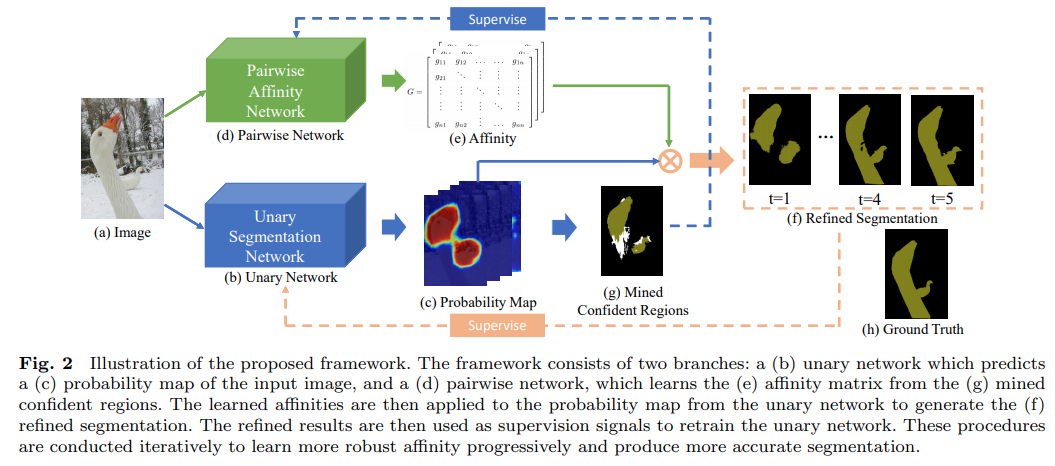
Fig. 97 Illustration of the proposed framework (source: arXiv:2002.08098)#
Unary network를 이용하여 추출한 probability map \(\alpha_t^u\)을 confident region mining을 거친 후 pairwise network의 supervision으로 사용함.
Pairwise Network를 이용하여 구한 affinity transformation matrix \(G\)를 이용하여 coarse label probabilities map \(\alpha_t^u\)를 refine 하여 \(\alpha_t^p=G_t\alpha_t^u\)를 구한 후 다음 step의 Unary network의 supervision으로 사용.
2. Formulation#
각 영상을 un-directed weighted graph \(\mathcal{G}=(V,E)\)로 표현함. → Vertex set \(V=\{v_1,...,v_N\}\)에서 vertex \(v_i\)와 \(v_j\)간의 edge는 weight \(w_{ij}\)를 갖는다 → 모든 weight는 adjacency matrix \(W=(w_{ij})_{i,j=1,...,N}\)로 표현 가능하다. → Degree matrix \(D\)는 degrees \(d_1,...d_N\)를 element로 갖는 diagonal matrix이다. → Degree는 \(d_i=\sum_{j=1}^{N}w_{ij}\)으로 표현된다.
Semantic segmentation을 위해 다음과 같은 energy loss function을 minimize 한다. (유사도가 높은 픽셀이 유사한 label을 같도록 학습함.)
\[\alpha^*=\arg\max_{\alpha}J(\alpha, W_f, W_g)=\arg\min_{\alpha}\alpha^{T}L\alpha\]여기서 \(\alpha\)는 output label의 hidden state, \(L=D-W\)는 Laplacian matrix이며 \(\alpha^TL\alpha\)는 다음과 같이 나타낼 수 있다.
\[\begin{split}\begin{aligned} \alpha^TL\alpha&=\alpha^T(D-W)\alpha \\ &=\sum_{i=1}^{N}d_i\alpha_i^2 - \sum_{i,j=1}^{N}\alpha_i\alpha_jw_{i,j} \\ &=\frac{1}{2}\left( \sum_{i=1}^{N}d_i\alpha_i^2-2\sum_{i,j=1}^{N}\alpha_i\alpha_j w_{i,j}+\sum_{j=1}^{N}d_j\alpha_j^2\right) \\ &=\frac{1}{2}\sum_{i,j=1}^{N}w_{i,j}(\alpha_i,\alpha_j)^2 \end{aligned}\end{split}\]본 논문에서는 \(\alpha\)를 추정하기 위해 similarity metric을 설계하고 최적화하는 방법을 사용하지 않고, EM formulation 기반으로하는 iterative learning method를 적용하여 probability map과 network를 refine하는 방법을 제안함.
\(G=I-D+W=I-L\)가 Pairwise network \(g\)와 pairwise/unary network의 output \(\alpha_p\)와 \(\alpha_u\)를 이용하여 학습가능한 Affinity transformation matrix를 나타낸다면, EM procedure는 다음과 같이 나타낼 수 있다.
(1) Initialization:
Class Activation Map에서 얻은 object seed \(Y_0\)를 이용하여 초기 parameter \(\{W_f,W_g\}_0\)과 unary response map \(\alpha_0^u\)를 얻는다.
(2) E-Step
\(t\) iteration에서의 \(J_t\) loss를 최소화하여 unary probability를 refine 하고,
\[\frac{\partial J}{\partial \alpha_t^u}=L_t\alpha_t^u=(1-G_t)\alpha_t^u\]\(t\)-step에서의 pairwise network의 output \(\alpha_{t}^{p}\)를 refine 한다.
\[\alpha_{t}^{p}=\alpha_{t}^{u}-\Delta\alpha_{t}^{u}\]unary probability map을 이용하여 pairwise network의 output을 refine한다.
\[\alpha_{t}^{p}=G_t\alpha_{t}^{u}\](3) M-Step
\(J_t\)가 최소화 되도록 Network \(f_{t+1}(x,W_f)\)와 \(g_{t+1}(x,W_g)\)의 parameter \(W_f\)와 \(W_g\)를 학습한다. 이때 supervision은 \(\alpha_{t}^{p}\)로부터 추출한다.
Energy function \(\mathbf{J}\)가 \(\alpha\)와 network parameter에 모두 미분 가능하기 때문에 EM procedure가 local minimum에 항상 수렴하는 것을 보이는 것은 간단함.
For training pairwise network in the \(t+1\) step, we consider the softmax cross-entropy loss function
\[H(\alpha_{t+1}^u)=-{\alpha_{t+1}^u}^{\top}\log\alpha_{t+1}^{P}\]여기서 \(\log\)함수가 단조 증가 함수이고, pairwise network의 output이 \(\alpha_{t+1}^p=G_{t+1}\alpha_{t+1}^u\)이므로
\[\begin{split}\begin{aligned} \min H&=-\min{\alpha_{t+1}^u}^{\top}\alpha_{t+1}^{P} \\ &=-\min \alpha_{t+1}^u G_{t+1}\alpha_{t+1}^u =-\min {\alpha_{t+1}^u}^{\top}(I-L_{t+1})\alpha_{t+1}^u \end{aligned}\end{split}\]to opt. \(\alpha_{t+1}^P\)is to min. the second term
\[L_{t+1}=\arg\max_{L_{t+1}^*}{\alpha_{t+1}^u}^{\top}L_{t+1}\alpha_{t+1}^u\]\(\alpha_t^{p}\)를 unary network와 \(\alpha_{t+1}^{u}\)을 학습하기 위한 supervision으로 사용하는 과정은 M-step으로 나타낼 수 있음.
하지만 pairwise network가 iteration을 반복하며 affinity를 학습하는 과정중에 정확한 supervision이 제공되지 않기 때문에 항상 수렴한고 말할 수 없음.
본 논문에서는 이런 문제를 해결하기 위해 mining된 confidence region \(Y_{t+1}\) 적용함. 이를 이용하면 energy function을 낮출 수 있을 것으로 예상함.
\[Y_{t+1}^{\top}L_{t+1}\alpha_{t+1}^u\leq {\alpha_{t+1}^u}^{\top}L_{t+1}\alpha_{t+1}^u\]
2. Training#
(1) Unary network
AffinityNet과 유사한 형태로 구성. DeepLab 구조에 object seed로 CAM을 사용함.
CAM으로부터 얻은 pseudo label을 이용하여 fully-supervised method와 같은 방법으로 softmax loss를 이용하여 학습함.
Unary network를 이용하여 추출한 probability map \(\alpha_t^u\)을 confident region mining을 거친 후 pairwise network의 supervision으로 사용함.
(2) Pairwise network
Spatial Propagation Network(SPN) 구조 적용하여 affinity transformation matrix \(G\)를 학습
정확한 label supervision이 주어지지 않는 상황이기 때문에 segmentation 결과가 정확하지 않아 학습이 잘 안될 수 있음
이 문제를 해결하기 위해 segmentation 결과로부터 confident region을 mining한 후 이를 이용하여 학습하는 과정을 거침. pairwise affinity network를 학습하기 위해 softmax loss를 적용함.
\[\mathcal{L}_{\alpha}=-Y_t^{\top}\log\alpha_t^p\]여기서 \(Y_t\)는 mining된 confidnet regions임.
잘 학습된 affinity matrix는 같은 object 내의 pixel들 처럼 같은 label을 가지는 영역에서는 smooth한 결과를 가지고, 명확한 경계를 가져야 함. 정확한 affinity matrix를 학습하기 위해, superpixel을 이용한 region smoothness loss를 제안함.
\[\mathcal{L}_s=||G-G_s||_2^2\]여기서 \(G\)는 affinity matrix를, \(G_s\)는 각 super-pixel region을 나타냄.
\(G\)를 이용하여 coarse label probabilities map \(\alpha_t^u\)를 refine 하여 \(\alpha_t^p=G_t\alpha_t^u\)를 구한 후 다음 step의 Unary network의 supervision으로 사용.
(3) Mining Confident Region
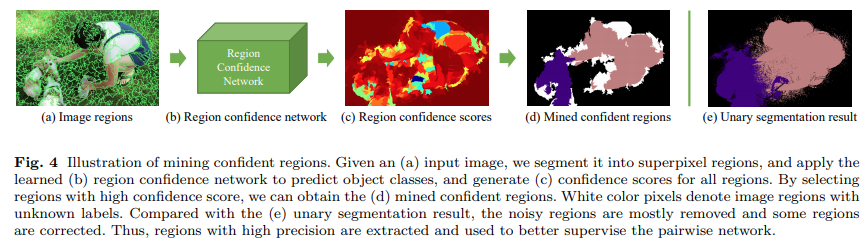
Fig. 98 Illustration of mining confident regions (source: arXiv:2002.08098)#
정확한 label annotation없이 신뢰할 수 있는 affinity matrix를 추출하기 위해 Confidence region을 mining함.
(1) image에서 분할한 각 super-pixel region \(S=\{S_{i,j}\}\)로 분할함.
(2) 어떤 super-pixel의 80% 이상이 unary network의 output에서 class \(c\)에 matching 된다면 이 superpixel을 class \(c\)의 sample로 사용함 \(\mathcal{D}=\{S,L\}_{i,j}\)
(3) 각 class의 sample을 region classification network \(f_c^m\)를 이용하여 confidence score를 구함.
(4) segment label과 다른 classification 결과가 나오는 영역을 제거함.
region classification network \(f_c^m\)는 mask pooling scheme을 포함하는 fast R-CNN구조를 적용하며, unary network의 label 정보를 supervision하는 cross-entropy loss을 이용하여 학습함.
\[\mathcal{L}_m=-\sum_{i,j,c}L_{i,j}(c)\log f_c^m(S_{i,j}|\theta_m)\]여기서 \(L_{i,j}\)는 one-hot vector로 class c 에 해당하는 element만 1의 값을 가짐.
Experimental Result#
1. Performance Evaluation#
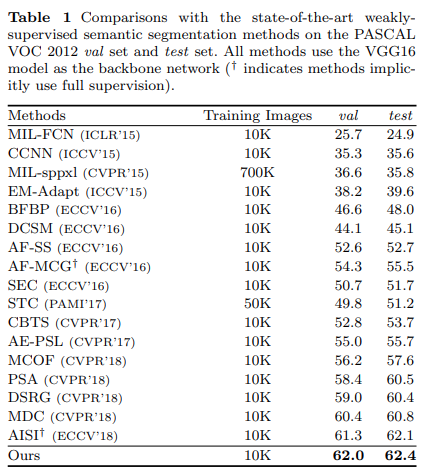
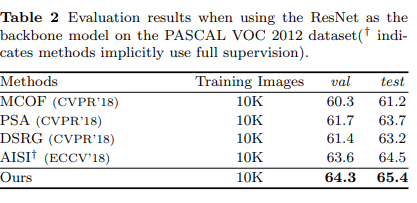
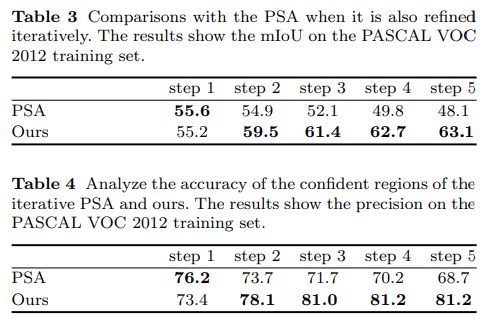
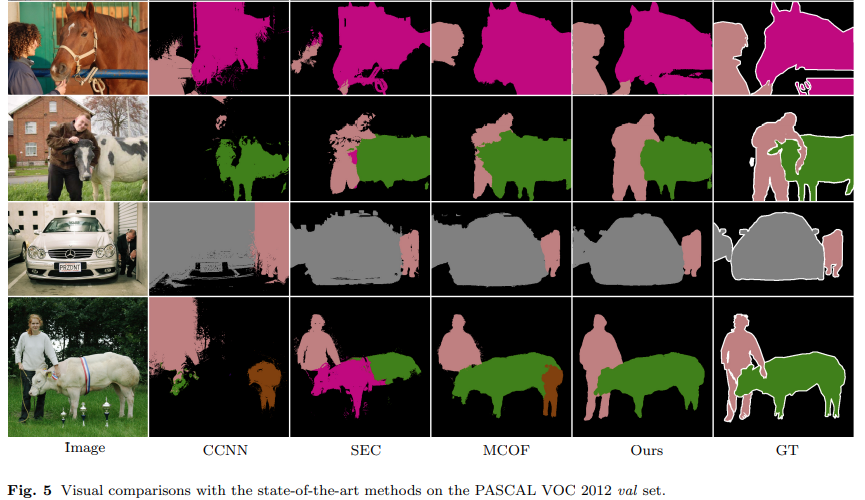
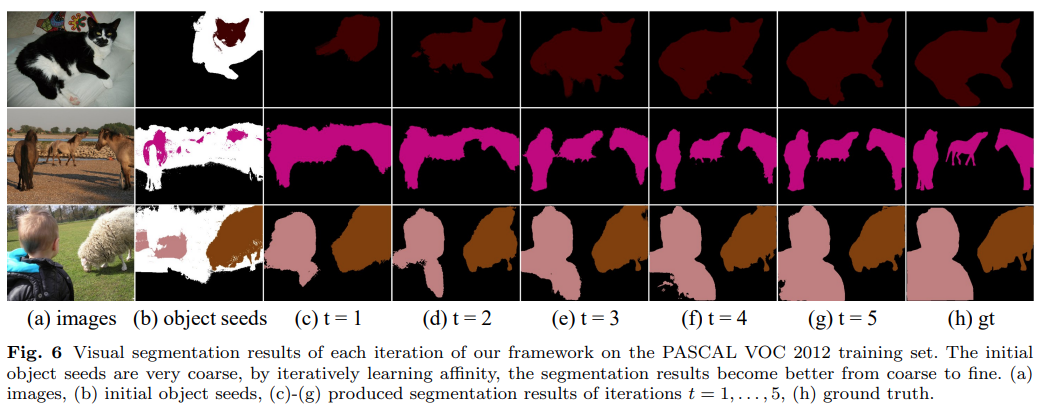