PanopticFCN - CVPR 2021
Contents
PanopticFCN - CVPR 2021#
Information
Title: End-to-End Object Detection with Transformers, CVPR 2021
Reference
Review By: Taeyup Song
Last updated on Nov. 1, 2022
Contribution#
Panoptic Segmentation의 things/stuff는 서로 다른 특성을 가지고 있음. Countable인 things class는 instance-aware features에 의존하며, object 주변에 위치한다. uncountable인 stuff class는 semantically consistent 특성, 즉 stuff class에 속한 pixel은 위치와 상관없이 같은 semantic을 가지면 같은 class로 구분되는 특성을 가짐.
Panoptic-FPN과 같은 instance(thing)/semantic(stuff) branch가 분리되어 있는 separate representation 구조와 다르게 통일된 구조로 panoptic segmentation을 수행하는 unified representation을 적용함.
각 instance 별로 kernel을 encode한 후 convolution을 통해 바로 prediction을 수행하는 구조를 제안하여 things와 stuff를 동일한 resolution에서 함께 prediction함.
Proposed Method#
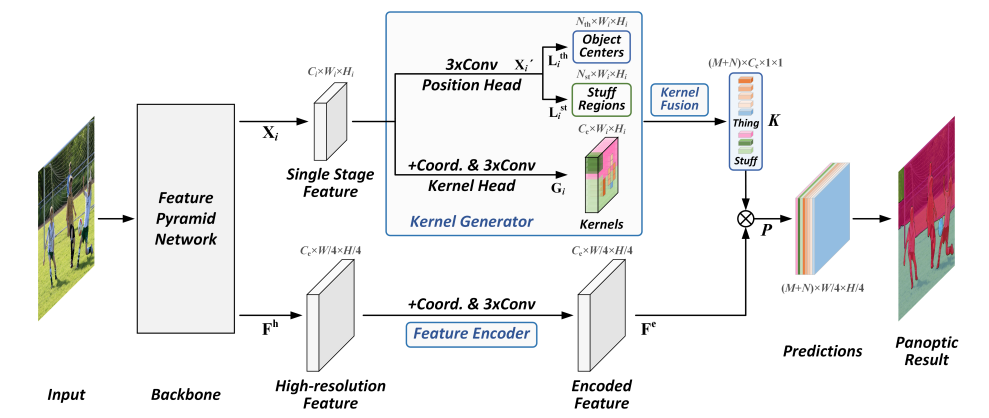
Fig. 82 The framework of Panoptic FCN (source: arXiv:2012.00720)#
1. Kernel Generator#
Kernel Generator는 CornerNet과 같은 point-based object detector와 유사한 구조 적용
FPN의 \(i\)번째 stage feature \(\mathbf{X}_i\)에서 position과 kernel weight를 생성하는 것을 목표로 함.
Position Head: things/stuff class의 position 정보 \(\mathbf{L}_i^{th}\in \mathbb{R}^{N_{th}\times W_i \times H_i}\), \(\mathbf{L}_i^{st}\in \mathbb{R}^{N_{st}\times W_i \times H_i}\)
Kernel Head: kernel weight map \(\mathbf{G}_i\in \mathbb{R}^{C_{e}\times W_i \times H_i}\)
(1) Position head
Things class가 주로 분포하는 object centers와 stuff region을 이용하여 각 category의 위치를 나타낸다. (각 feature position에 대해 어떤 class에 속하는지 나타냄.)
Things class: \(k\)번째 object가 things class중 class \(c\)에 해당한다고 할 때, heatmap \(\mathbf{Y}_i^{th}\in[0,1]^{N_{th}\times W_i \times H_i}\)에서 object에 해당하는 region의 \(c\)번째 channel에 1이 assign된다.
Stuff class: \(\mathbf{Y}_i^{st}\in[0,1]^{N_{st}\times W_i \times H_i}\)에 각 class에 해당하는 channel에 one-hot semantic label이 영역 크기에 맞게 표기됨.
같은 semantic을 가지는 배경 영역은 하나의 instance로 취급한다.
Position head를 학습하기 위한 loss function은 thing class에 대한 object center loss \(\mathcal{L_{pos}^{th}}\)와 stuff regions에 대한 loss \(\mathcal{L}^{st}_{pos}\)의 합으로 표현된다.
\[\begin{split} \begin{aligned} \mathcal{L}_{\text{pos}}^{\text{th}}&=\sum_i\text{FL}(\mathbf{L}_i^{\text{th}},\mathbf{Y}_i^{\text{th}})/N_{\text{th}}, \\ \mathcal{L}_{\text{pos}}^{\text{st}}&=\sum_i\text{FL}(\mathbf{L}_i^{\text{st}},\mathbf{Y}_i^{\text{st}})/W_{i}H_{i},\\ \mathcal{L}_{\text{pos}}&=\mathcal{L}_{\text{pos}}^{\text{th}}+\mathcal{L}_{\text{pos}}^{\text{st}} \end{aligned} \end{split}\]여기서 \(FL()\)은 focal loss이며, \(N_{th}\) 와 \(N_{st}\) 는 각각 things와 stuff의 semantic cetegory의 수이다.
(2) Kernel head
Kernel head에서는 먼저 spatial cues를 확보하기 위해 CoordConv.를 적용하여[ref, ref] 유사하게 feature \(X_i\)의 상대적인 좌표 정보(xx, yy)를 concat하여 → \(X_i^{''}\in \mathbb{R}^{(C_i+2)\times W_i \times H_i}\)
3개의 Conv. layer를 거쳐 Kernel weight map \(\mathbf{G}_i \in \mathbb{R}^{C_e \times W_i \times H_i}\) 를 생성한다.
position head로 부터 predictions \(D_i^{\text{th}}\)와 \(D_i^{\text{st}}\)가 주어지면, kernel weights는 대응되는 instances를 표현하도록 선택됨.
e.g. things category \(c\)에 속한 pixel \((x_c, y_c)\in D_{i}^{\text{th}}\)에 대응되는 kernel weight \(\mathbf{G}_{i,:,x_c,y_c} \in \mathbb{R}^{C_e \times 1 \times 1}\)은 category \(c\)를 추정하도록 값이 설정됨.
2. Kernel Fusion#
기존 연구[39, 12, 45]에서는 후처리 과정에서 NMS를 적용함
본 논문에서는 kernel fusion operation을 이용하여 things class의 instance awareness와 stuff class의 semantic consistency를 보전하며 다수의 FPN stage에서 반복적으로 생성되는 kernel weights를 merge한다.
\[ K_i=\text{AvgCluster}(G'_j), \]여기서 \(\text{AvgCluster}()\)는 average-clustering operation이며, candidate set \(G'_j=\{G_m:\text{ID}(G_m)=\text{ID}(G_j)\}\) 는 예측 결과의 ID가 \(G_j\) 과 같은 모든 kernel weight의 평균을 구한다.
연산 과정에서 동일한 ID를 가지는 kernel weight를 하나로 merge하여 things class의 개별 instance와 staff class의 semantic categories를 각각 단일 kernel로 표현한다. 따라서 things class에 대한 instance-awareness와 stuff class에 대한 semantic-consistency를 동시에 만족하는 결과를 얻을 수 있다.
3. Feature Encoder#
Instance의 representation의 details를 보전하기 위해, FPN에서 생성된 고해상도 feature \(\mathbf{F }^h\in \mathbb{R}^{C_e \times W/4 \times H/4}\) 를 feature encoding에 적용한다.
Feature \(\mathbf{F}\) 가 주어지면 coord conv. 및 convolution 연산을 적용하여 position cues가 encoding된 feature \(\mathbf{F}^e\in \mathbb{R}^{C_e \times W/4 \times H/4}\) 를 생성한다.
things와 stuff에 대한 kernel weight \(K^{th}\) 와 \(K^{st}\)가 각각 \(M\), \(N\) 개 주어지면, 각 instance는 다음 연산을 통해 생성된다.
\[ \mathbf{P}_j=K_j \otimes \mathbf{F}^e \]여기서 \(\otimes\) 는 convolution 연산을 나타낸다.
즉 생성된 kernel weight에 대해 위 연산을 수행하면, \(W/4 \times H/4\) 해상도의 M+N개의 instance prediction을 생성한다. 생성된 instance prediction은 PanopticFPN과 동일하게 원본 크기로 resize 후 pixel별 class를 추정한다.
4. Training and Inference#
Training Scheme
학습 과정에서 각 object의 center point와 stuff regions의 모든 points는 things/stuff를 위한 kernel weights 생성에 활용되어진다.
Segmentation을 예측하기 위해 [Dice Loss]를 적용한다.
\[ \mathcal{L}_{\text{seg}}=\sum_j\text{Dice}(\mathbf{P}_j,\mathbf{Y}_j^{\text{seg}})/(M+N), \]여기서 \(\mathbf{Y}_j^{seg}\)는 j번째 예측 \(\mathbf{P}_j\)에 대응되는 ground truth이다.
Kernel generator를 더 잘 학습시키기 위해 각 object에 대해 다수의 positive sample을 sampling 하여 사용한다. 각 object \(\mathbf{L}_i^{\text{th}}\)의 내부에서 top prediction score \(s\)를 가지는 \(k\)개의 position을 선택하여 각 instance별로 \(k \times M\) kernels을 생성한다.
stuff region의 경우 동일한 class/category에 속한 모든 point를 동등하게 반영하기 위해 factor \(k\)를 1로 설정한다.
본 논문에서는 original Dice Loss를 weighted version으로 수정하여 사용한다.
\[ \text{WDice}(\mathbf{P}_j,\mathbf{Y}_j^{\text{seg}})=\sum_k w_k\text{Dice}(\mathbf{P}_{j,k},\mathbf{Y}_{j}^{\text{seg}}), \]여기서 \(w_k=\frac{s_k}{\sum_i s_i}\)으로 \(k\)번째 weighted score를 나타낸다.
최적화 target loss \(\mathcal{L}\)은 position head loss \(\mathcal{L}_{pos}\)와 weighted Dice Loss의 weighted sum으로 표현된다.
Inference Scheme
Inference 커널생성 후 segmentation을 수행한다.
Step 1) \(i\) 번째 position head로 부터 stuff, thing class에 해당하는 feature position을 aggregate
Step 2) MaxPooling을 이용하여 Object center에 해당하는 peak point를 보전함
Step 3) Kernel fusion 과정을 통해 top 100 score를 가지는 kernel을 생성하고 Feature encoder의 output과 conv 연산을 통해 prediction \(\mathbf{P}\) 를 구함
Step 4) Threshold 0.4를 적용하여 softmask 생성하고, argmax를 적용하여 겹치지 않는 panoptic result를 도출함.
Experimental Result#
1. Experimental Setting#
Backbone and feature extractor: ResNet with FPN (P2 to P5)
Dataset:
COCO (80 thins class, 53 stuff class)
Cityscape
Mapillary Vistas (37 things, class, 28 stuff class)
2. Result#
COCO validation set에서 deformable conv.등 간단한 개선 과정을 거친 모델 (Panoptic FCN*)이 기존 방법 대비 가장 높은 PQ를 나타냄을 알 수 있다.
또한 입력 크기를 400pixel로 했을 때 (Panoptic FCN-400) 비교적 높은 성능을 유지하면서 가장 높은 FPS를 나타냄을 알 수 있다.
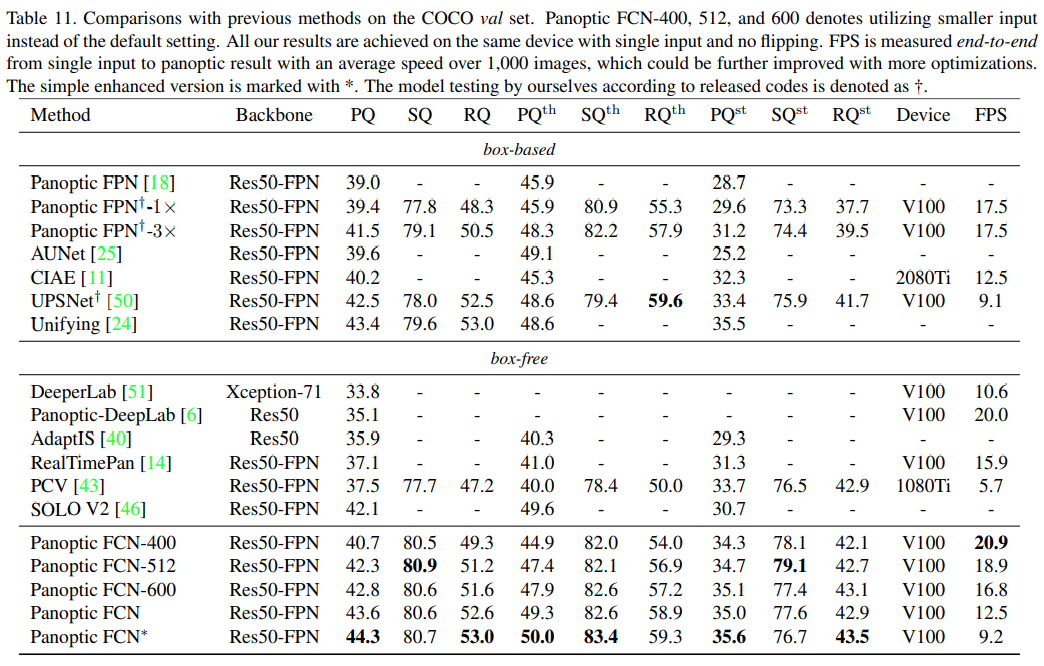
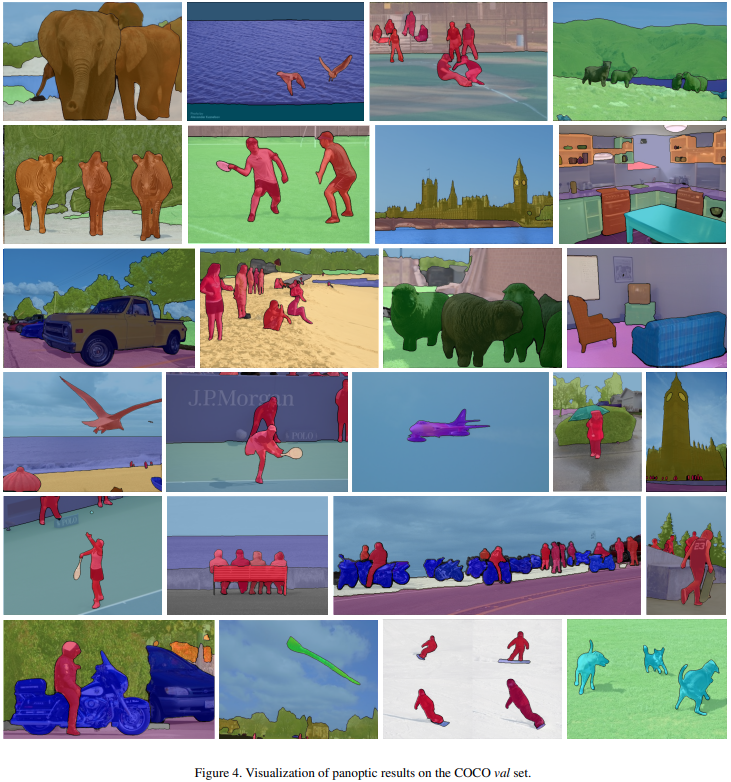