SEAM - CVPR 2020
Contents
SEAM - CVPR 2020#
Information
Title: Self-supervised Equivariant Attention Mechanism for Weakly Supervised Semantic Segmentation, CVPR 2020
Reference
Paper : https://arxiv.org/abs/2004.04581
Review By: 김현우 (Kim Hyeonwoo)
Last updated on Aug. 16, 2022
Abstract#
Image-level supervision을 이용한 Weakly-supervised Semantic Segmentation (WSSS)의 대부분은 Class Activation Map (CAM)을 이용한 접근을 사용한다. 하지만, CAM의 경우는 full-supervision과 weak-supervision의 차이로 인해 object mask를 cover 하기에는 문제가 있다.
본 논문은 추가적인 supervision의 제공과 full-supervision과 weak-supervision 사이의 차이를 줄일 수 있는 Self-supervised equivariant attention mechanism (SEAM)을 제안함.
Semantic segmentation의 경우 spatial transformation에 상관없이 같은 pixel에 일관성 있는 클래스를 가지도록 함 (equivariance)
하지만, 이러한 제약은 image-level을 통해 CAM을 생성할 때 없어지기 때문에 various transformed images에 consistency regularization을 적용하는 방법을 제안함. 이를 통해 transformation에 따라서 CAM의 결과가 달라지지 않도록 regularization을 제공
추가적으로 pixel correlation module (PCM)을 이용하여 다른 픽셀의 정보를 통해서 CAM의 성능을 향상시킴
PASCAL VOC 2012 dataset에서 SOTA 성능을 달성
Problem Statement#
일반적으로, 대부분의 WSSS 방법의 경우는 class activation map (CAM)이라는 효율적으로 image classification labels을 이용하여 class에 따른 object의 영역을 추정하지만, CAM의 attention이 object의 discriminative가 가장 높은 영역에만 생기거나(under-activation), 배경영역에도 attention이 나타나는 (over-activation) 문제점이 있음. (부정확함.)
또한 생성된 CAMs은 affine transformations 적용했을 때, 일관성 있는 결과를 보이지 않음.
Fig. 115 CComparisons of CAMs generated by input images with different scales. (source: arXiv:2004.04581)#
Fig. 115와 같이 rescaling transformations에 따라서 CAM의 attention region이 변함을 확인할 수 있다. 이러한 현상의 근본적인 원인은 fully and weakly supervision 사이에서 오는 차이에 의해서 발생한다.
Contribution#
다양한 Image transformation에 대응하는 consistency regularization을 통해서 self-supervision을 제공하는 self-supervised equivariant attention mechanism (SEAM) architecture를 제안.
네트워크의 예측 일관성을 높이기 위해, pixel correlation module (PCM)을 통해 각 픽셀을 위한 context appearance information를 잡고 학습된 affinity attention maps에 의해서 CAMs을 개선
SEAM은 CAMs과 revised CAMs 간의 차이를 최소화하는 equivariant cross regularization (ECR) loss를 통해서 siamese network를 학습.
PCM과 self-supervision이 효율적으로 결합되어 over-activated and under-activated regions을 줄임
PASCAL VOC 2012에서 SOTA를 달성
Proposed Method#
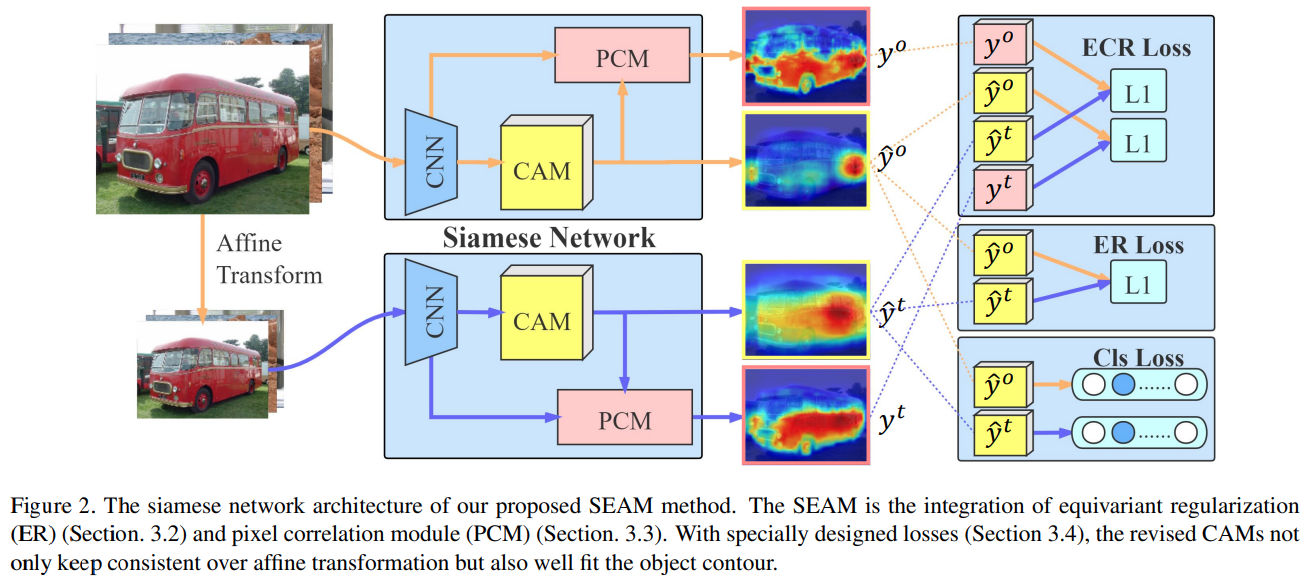
Fig. 116 The siamese network architecture of our proposed SEAM method. (source: arXiv:2004.04581)#
1. Motivation#
Parameter \(w_s\)를 가지는 semantic segmentation function이 \(F_{w_s}(\cdot)\)와 parameter \(w_c\)를 가지는 classification function이 \(F_{w_c}(\cdot)\)일 때, WSSS에서는 최적의 segmentation과 classification parameter가 \(w_s=w_c\)를 만족한다는 가정을 기반으로 한다.
하지만 segmentation 함수는 equivariant에 치중하는 반면, classification은 pooling operation에 의해서 invariance에 치중하는 경향을 나타내기 때문에 학습 과정에서 두 task의 objective를 모두 달성하는 것은 불가능하다.
Affine transformation \(A(\cdot)\)을 각 sample에 적용한다고 가정하면, segmentation function은 equivariant 경향을 나타낸다.
\[ F_{w_s}(A(I)) = A(F_{w_s}(I)) = s \]여기서 \(s\)는 pixel-level segmentation mask를 나타낸다.
Classification function은 invariance에 초점을 맞춘다. 이 특성은 pooling 연산이 주된 요인이다.
\[ Pool(F_{w_c}(A(I))) = Pool(F_{w_c}(I)) \]Self-attention은 network의 성능 개선을 위해 널리 사용되고 있으며, context feature dependency를 통해서 feature map을 개선할 수 있다. Aactivation map을 개선하기 위해 pixels간의 유사도를 이용하는 대부분의 WSSS 방법과 유사한 아이디어라고 할 수 있다.
일반적인 self-attention은 다음과 같은 수식으로 표현할 수 있다.
\[ y_i=\frac{1}{\mathscr{C}(x_i)}\sum_{\forall j}f(x_i,x_j)g(x_j)+x_i \]\[ f(x_i, x_j)=e^{\theta(x_i)^T \phi(x_j)} \]여기서 \(g(x_j)\)는 input signal \(x_j\)의 representation, \(f(x_i, x_j)\)는 embedding space에서 계산되는 dot-produnct pixel affinity를 나타낸다. Output signal은 \(C(x_i)=\sum_{\forall j}f(x_i, x_j)\)를 이용하여 normalize된다.
제안된 SEAM구조는 network가 일관적인 결과를 출력할 수 있도록 self-attention을 적용한다.
2. Equivariant Regularization (ER)#
Fully supervised semantic segmentation 에서는 data augmentation에 의해 pixel-level labels의 변화가 생기지 않으며, 네트워크에는 implicit한 equivariant regularization이 생긴다.
그러나, WSSS는 이미지 레이블을 통해 분류만 하기에 이러한 제약이 없기 때문에 같은 ER loss를 제안함.
\[ L_{ER} = ||F(A(I))-A(F(I))||_{1} \]여기서 \(F\) network, \(A\)는 rescaling, rotation, flip과 같은 Affine Transformation을 나타낸다.
→ Affine Transformation을 적용한 Image에서 추출한 CAM과 Original Image에서 추출한 CAM에 Affine Transformation을 적용한 CAM 사이의 차이를 최소화함으로서, CAM간의 일관성을 보장함. (ER Loss)
3. Pixel Correlation Module (PCM)#
ER Loss가 추가적인 supervision을 제공하지만, classical convolution layers 만으로는 이상적인 equivariance를 이루기 어렵다.
이를 해결하기 위해서 self-attention에서 제공하는 context information 이용하여 pixel-wise prediction 결과를 개선한다.
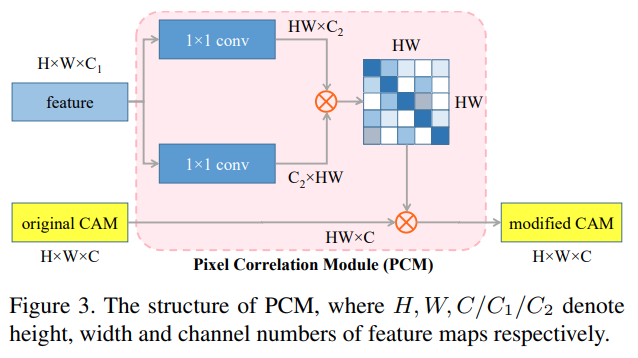
Fig. 117 The structure of PCM. (source: arXiv:2004.04581)#
Final CAM의 경우 original CAM과 normalized similarities을 적용한 CAM을 가중합하여 계산한다.
고전적인 self-attention과는 다르게, PCM의 경우 residual connection을 제거해서 original CAM과 같은 강도를 유지한다.
다른 네트워크 브랜치는 PCM에 대한 픽셀 수준의 supervision을 제공하지만 이는 ground truth만큼 정확하지 않기 때문에 \(\phi, g\) 의 embedding function을 제거해서 파라미터의 수를 줄임으로서 부정확한 supervision에 대한 overfitting을 방지한다.
4. Loss Design of SEAM#
제안된 논문은 WSSS 조건에 의해 Image-level classification label \(l\) 만 supervision으로 학습에 사용한다.
(1) Multi-label soft margin loss for Multi-class classification
Siamese network의 original CAM, 즉 원본 영상으로부터 구한 CAM \(\hat{y}^{o}\)와 transformed image에서 구한 CAM \(\hat{y}^{t}\) 에 대해 global average pooling(GAP) layer를 도입해서 이미지 분류를 위한 prediction vector \(z\)를 생성하고, multi-label soft margin loss를 통해서 네트워크를 학습한다.
두 branch에 대한 classification loss는 다음과 같이 정의된다.
\[\begin{split} \begin{aligned} L_{cls} &= \frac{1}{2}(l_{cls}(z^o,l) + l_{cls}(z^t,l)) \\ &\text{where }l_{cls}(z,l) = -\frac{1}{c} \sum_{c=1}^{C-1}[l_{c}log(\frac{1}{1+e^{-x_c}}) + (1-l_c)log(\frac{e^{-x_c}}{1+e^{-x_c}})] \end{aligned} \end{split}\]여기서 \(l_{cls}\) 는 \(C-1\) 개의 foreground object category에 대한 classification loss이다.
(2) ER (Equivariant Regularization) loss
(ER) loss는 다음과 같이 정의된다.
\[ L_{ER} = ||A(\hat{y^o})-\hat{y^t}||_{1} \]여기서 \(A(\cdot)\)은 transformation branch에서 input image에 대해 적용되는 affine transformation이다.
(3) ECR (Equivariant Cross Regularization) loss
CAM을 개선하기 위해 두 branch로 구한 CAM에 PCM 모듈을 적용하는데, 실험 결과 학습 과정에서 쉽게 local minima에 빠져 모든 pixels을 동일한 class로 predict하는 문제가 발생했다.
이런 문제를 해결하기 위해 ECR loss를 제안한다.
\[ L_{ECR} = ||A({y^o})-\hat{y^t}||_{1} + ||A(\hat{y^o})-y^t)||_{1} \]PCM의 output은 original CAM에 의해 regularize된다. 이를 통해 PCM refinement 적용중에 CAM이 degeneration되는 것을 방지할 수 있다.
(4) Background score
CAM의 경우는 foregorund class에 대해서 정의되지만, 입력 이미지의 대부분의 경우는 background 를 가지기에 PCM 과정에서 이를 무시할 수 없다.
따라서, background positions에 zero vectors를 추가해서 PCM 계산에는 포함하고, gradients는 전파하지 않는다.
\[ \hat{y}_{i,bkg} = 1 - \max_{1\le c \le {C}}{\hat{y}_{i,c}} \]# 배경 인덱스 0을 무시하고 계산하는 것을 확인할 수 있음 # label = [배경, 개, 고양이] loss_cls1 = F.multilabel_soft_margin_loss(label1[:,1:,:,:], label[:,1:,:,:]) loss_cls2 = F.multilabel_soft_margin_loss(label2[:,1:,:,:], label[:,1:,:,:]) ns,cs,hs,ws = cam2.size() loss_er = torch.mean(torch.abs(cam1[:,1:,:,:]-cam2[:,1:,:,:])) #loss_er = torch.mean(torch.pow(cam1[:,1:,:,:]-cam2[:,1:,:,:], 2)) <- cam1[:,0,:,:] = 1-torch.max(cam1[:,1:,:,:],dim=1)[0] cam2[:,0,:,:] = 1-torch.max(cam2[:,1:,:,:],dim=1)[0] tensor_ecr1 = torch.abs(max_onehot(cam2.detach()) - cam_rv1)#*eq_mask tensor_ecr2 = torch.abs(max_onehot(cam1.detach()) - cam_rv2)#*eq_mask loss_ecr1 = torch.mean(torch.topk(tensor_ecr1.view(ns,-1), k=(int)(21*hs*ws*0.2), dim=-1)[0]) loss_ecr2 = torch.mean(torch.topk(tensor_ecr2.view(ns,-1), k=(int)(21*hs*ws*0.2), dim=-1)[0]) loss_ecr = loss_ecr1 + loss_ecr2 loss_cls = (loss_cls1 + loss_cls2)/2 + (loss_rvmin1 + loss_rvmin2)/2 loss = loss_cls + loss_er + loss_ecr
Experiments#
1. Implementation Details¶#
Dataset: PASCAL VOC 2012 Benchmark (20 foreground objects and the background)
Data augmentation
SBD additional annotations [14] 를 적용하여 10582 images로 구성된 training set을 사용
Backbone
ResNet38 (output stride =8, 3,4번째 stage에서 feature map 추출하고 1x1 conv.를 통해 각각 64, 128 ch로 변환
2. Ablation Study#
Baseline과 비교한 결과 제안한 방법이 더 높은 mIoU를 나타냄을 확인하였고, SEAM을 이용하여 CAM을 생성한 결과 GradCAM보다 높은 성능을 확인할 수 있다.
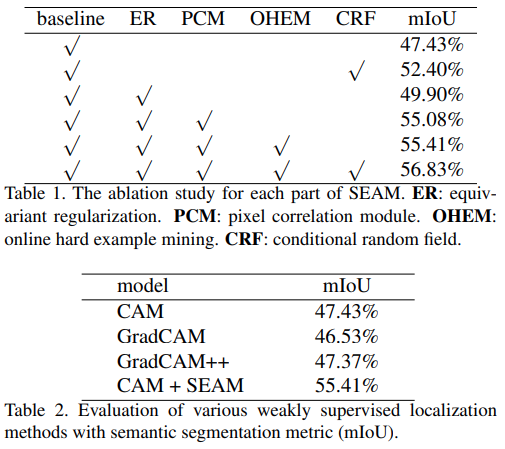
Improved Localization Mechanism:
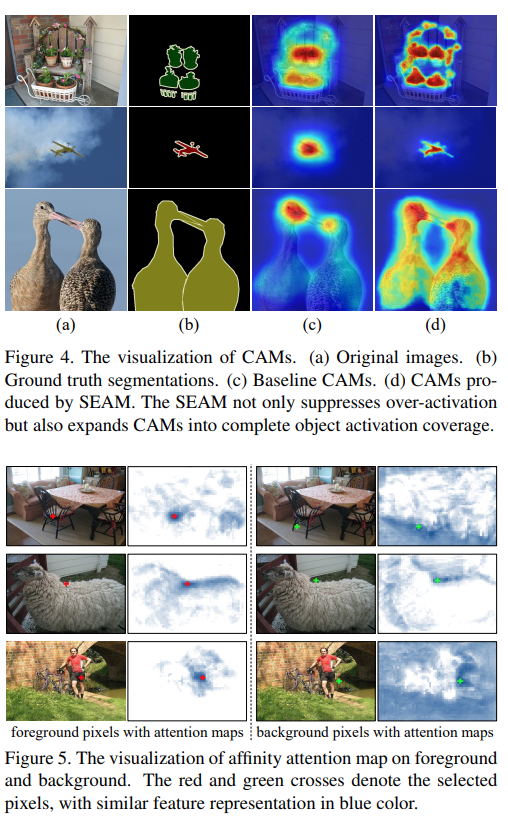
다양한 Affine Transformation에 대해 ER 방법의 효과를 검증한 결과 rescale만 단독 사용한 것이 가장 효과적임을 확인할 수 있다. (flip, rotation 등의 annotation을 함께 적용한 결과 성능 개선이 적음)
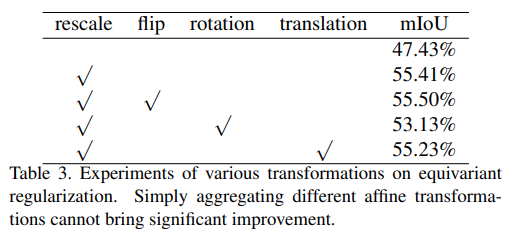
Augmentation and Inference:
Baseline의 rescaling 범위를 확장해도 pseudo label performance가 개선되지 않음. → ER과 PCM의 조합이 annotation의 효과가 아님을 알 수 있다. (Table 4)
Inference 과정에서 다른 스케일의 이미지에서 prediction을 aggregating하여 multi-scale test를 수행한 결과 baseline 대비 개선된 성능을 나타냄. (Table 5)
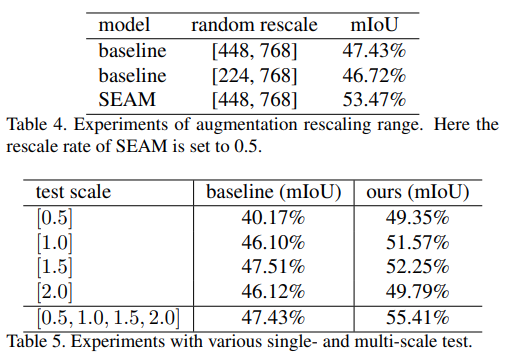
제안된 SEAM이 baseline 대비 낮은 mFN과 mFP을 나타냄을 알 수 있음. overactivated된 baseline CAM에 비해 object region을 잘 activation한다고 할 수 있다.
Image scale의 변화에 따라 mFN과 mFP의 변화가 존재하는 baseline 대비 제안된 SEAM은 일정한 수치를 나타내는 것을 확인할 수 있음. 이는 equivariance regularization이 network 학습 과정에서 효과를 나태네어 CAM을 개선한 것으로 해석할 수 있다.
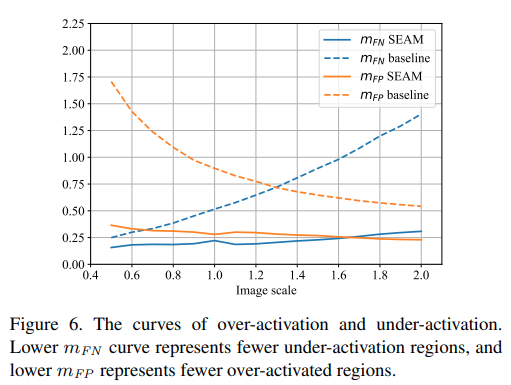
(3) Comparison with State-of-the-arts
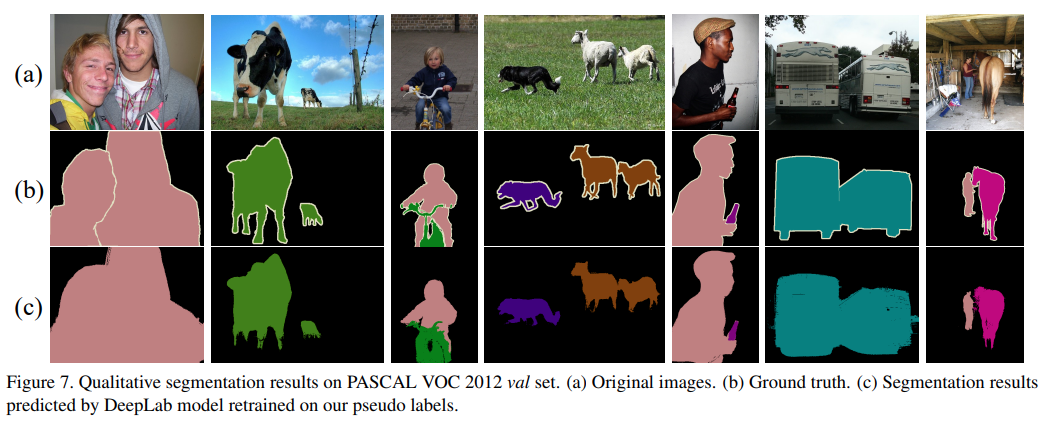

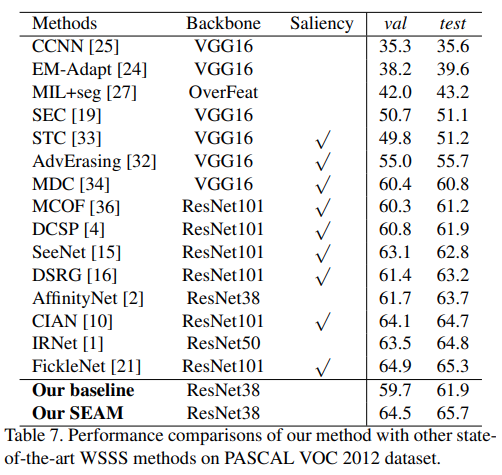
Conclusion#
In this paper, we propose a self-supervised equivariant attention mechanism (SEAM) to narrow the supervision gap between fully and weakly supervised semantic segmentation by introducing additional self-supervision
The SEAM embeds self-supervision into weakly supervised learning framework by exploiting equivariant regularization, which forces CAMs predicted from various transformed images to be consistent.
To further improve the ability of network for generating consistent CAMs, a pixel correlation module (PCM) is designed, which refines original CAMs by learning inter-pixel similarity
Our SEAM is implemented by a siamese network structure with efficient regularization losses. The generated CAMs not only keep consistent over different transformed inputs but also better fit the shape of ground truth mask
The segmentation network retrained by our synthesized pixel-level pseudo labels achieves state-of-the-art performance on PASCAL VOC 2012 dataset, which proves the effectiveness of our SEAM.