2.4. Recovery of affine and metric properties from images#
The Method to recover similarity properties from projective distored images#
평면에 projection되어 있는 이미지에서 사영 왜곡을 제거하면 각도, 길이 비율과 같은 similarity 속성을 원래 평면에서 측정할 수 있으며, 4개의 대응점을 지정하여 dof가 8인 projective transformation H를 계산해 사영 왜곡을 완전히 제거할 수 있습니다.
그러나 similarity transformation은 projective tranformation에 비해 dof가 4개 더 적습니다. 따라서 4 dof만 지정하여도 similarity 속성을 측정할 수 있습니다.
사영 왜곡된 이미지에서 similarity 속성을 복원하기 위한 4개의 dof는 line at infinity \(l_\infty\)(dof 2)와 \(l_\infty\)에 있는 두 개의 원형점(\(circular\ points\), dof 2)이라는 물리적 실체로 주어지며, 이러한 연관성을 이용하는 것이 행렬 분해에서 나타나는 행렬을 지정하는 방식보다 문제를 더 직관적으로 해결할 수 있습니다.
이미지에서 \(l_\infty\)가 결정되면 사영 왜곡을 제거할 수 있고, \(circular \ points\)가 결정되면 아핀 왜곡을 제거할 수 있습니다. 그러면 남은 왜곡은 similarity 뿐이므로, 이미지에서 similarity 속성을 측정할 수 있습니다.
2.4.1 The line at infinity#
사영 변환에 의해 왜곡된 이미지에서 무한선(the line at infinity) \(l_\infty\)을 식별하면 아핀 속성을 복원할 수 있습니다. 그 이유는 line at infinity \(l_\infty\)이 projective transformation H에 invariant하기 때문입니다.
2.4.2 Recovery of affine properties from images#
사영 변환에 의해 왜곡된 이미지에서 line at infinity \(l_\infty\)을 식별하여 line at infinity의 표준 위치인 \((0, 0, 1)^T\)로 보내는 변환을 적용하면, Result 2.17.에 의해 이미지에 적용된 변환은 아핀 변환임을 보일 수 있습니다.
즉 이미지의 아핀 속성을 복원한 것입니다. 다음 그림에서 이 핵심 아이디어에 대해 잘 설명하고 있습니다. 그렇다면 사영 변환에 의해 왜곡된 이미지에서의 line at infinity \(l_\infty\)을 식별하는 방법은 무엇이 있을까요?
이 책에서는 2가지 방법을 소개합니다.
평행선의 교점을 이용한 방법
길이 비율을 이용한 방법
위 두 가지 중 저희는 1번 방법에 대한 실습을 함께 제공합니다.
Affine rectification#
아래 이미지는 3가지의 평면과 그 사이에서의 일어날 수 있는 2가지 변환으로 아핀 속성이 보정되는 과정을 보입니다. 3가지 평면은 왼쪽부터 순차적으로 아래와 같은 평면을 나타냅니다.
실제 세계에서 왜곡 없이 평면으로 사영된 평면 \(\pi_1\),
projective transformation에 대해 변환되어 사영된 평면 \(\pi_2\),
\(\pi_2\)에서 아핀 속성을 복원한 \(\pi_3\)
또한, \(H_P\)는 projective transformation, \(H_A\)는 Affine transformation 입니다.
위 그림에서 알 수 있는 것은 2가지입니다.
projective transform에 의해 왜곡된 이미지에서 line at infinity \(l_\infty\)을 식별하여 affine 속성을 복원해 평행인 것을 평행으로 만들 수 있다는 것
위와 같이 복원된 평면은 원본과 affine transforation의 관계를 가진다는 것
The Method using the intersection of parallel lines#
실세상 평면의 line at infinity는 원근 이미지에서 평면의 vanishing line이 됩니다. 다음 그림에서 나와있듯이, vanishing line \(l\)은 이미지에서 평행선의 교점에서 계산할 수 있습니다.
vanishing line을 식별하는 방법을 요약해보겠습니다.
실제 세계에서는 평행한 두 직선을 2 pair 찾습니다.
외적을 이용해서 각 쌍에 존재하는 두 직선의 접점을 계산합니다.
위 과정을 통해 구한 2개의 접접의 외적을 이용해 선의 좌표 계산합니다.
아래 실습 코드가 있습니다.
import numpy as np
import cv2
import matplotlib.pyplot as plt
img = cv2.imread('./figures/chessboard.jpg', cv2.IMREAD_COLOR)
st = [np.array([1381, 969]), np.array([1719, 392]), np.array([337, 711]), np.array([1382, 968])]
ed = [np.array([338, 711]), np.array([786, 195]), np.array([786, 194]), np.array([1719, 392])]
# Homogeneous vectorize
st = np.append(st, np.ones((4,1), dtype=np.int32), axis=1)
ed = np.append(ed, np.ones((4,1), dtype=np.int32), axis=1)
# Compute vanishing point Vx
Vx = np.cross(np.cross(st[0], ed[0]),np.cross(st[1], ed[1]))
Vx = Vx/Vx[2]
# Compute vanishing point Vy
Vy = np.cross(np.cross(st[2], ed[2]),np.cross(st[3], ed[3]))
Vy = Vy/Vy[2]
# Compute vanishing line Vl
Vl = np.cross(Vx,Vy)
Vl = Vl/Vl[2]
print('Vanishing line : ', Vl)
# compute affine rectification matrix
H_A = np.eye(3)
H_A[2] = Vl
# recover the affine properties
recovered_img = cv2.warpPerspective(img, H_A, (0,0))
concat = np.concatenate([img, recovered_img], axis=1)
plt.imshow(concat)
Vanishing line : [6.61317266e-07 2.85906628e-04 1.00000000e+00]
<matplotlib.image.AxesImage at 0x168e874d0>
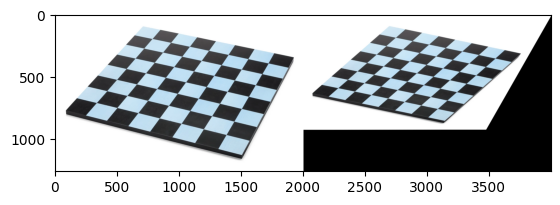
Computing a vanishing point from a length ratio#
또는 길이 비율이 알려진 두 개의 간격이 주어지면 소실점을 결정할 수 있습니다. 일반적인 경우는 이미지의 한 직선에서 세 점이 식별된 경우입니다. \(a, b, c\)가 실세계에서 대응하는 같은 선상의 점이고, 길이 비율 \(d(a,b) : d(b, c) = a : b\)가 알려져 있다고 가정합니다. 교차 비율을 사용하면 소실점을 찾아낼 수 있습니다.
이미지에서 거리 비율 \(d(a', b') : d(b', c') = a' : b'\)을 측정합니다.
점 \(a, b, c\)를 직선 \(<a, b, c>\)상의 좌표계에서 좌표 \(0, a, a + b\)로 표기합니다. 계산을 위해 이러한 점들을 2차원 동차 벡터 \((0, 1)^T, (a, 1)^T, (a+b, 1)^T\)로 표시합니다. 같은 방법으로 \(a', b', c'\)는 좌표 \(0, a', a'+b'\)을 가지고 동차 벡터로 표시할 수 있습니다.
위의 두 좌표계를 이용해 \(a\mapsto a', b\mapsto b', c\mapsto c'\)으로 가는 1차원 사영변환 \(H_\mathbf{2x2}\)를 계산합니다.
\(H_\mathbf{2x2}\)에 의한 무한점의 이미지가 \(<a', b', c'>\)의 소실점이 됩니다.
2.4.3 The circular points and their dual#
사영 왜곡된 이미지에서 원형점(the circular points) \(I, J\)를 식별하면 닮음 속성을 복원할 수 있습니다. 이는 원형점 \(I, J\)가 사영 변환 H에 고정된다는 것이 H가 닮음 변환인 것과 필요충분조건이기 때문입니다. 일반적으로 I와 J의 좌표는 아래와 같습니다.
\(\text{I} = \begin{pmatrix} 1 \\ i \\ 0 \end{pmatrix}, \ \ \ \ \ \ \ \ \text{J} = \begin{pmatrix} 1 \\ -i \\ 0 \end{pmatrix}.\)
아래 그림은 circular points가 similarity transformation에 invariant 하다는 것을 보여줍니다.
The conic dual to the circular points#
사영 왜곡된 이미지에서 circular points에 대한 dual conic \(C^*_\infty\)를 식별하여도 similarity 속성을 복원할 수 있습니다. 이는 \(C^*_\infty\)가 projective transformation H에 고정된다는 것이 H가 similarity transformation인 것과 필요충분조건이기 때문입니다.
※ circular points에 대한 duality conic \(C^*_\infty\)는 다음과 같이 주어집니다.
\(C^*_\infty = IJ^T + JI^T\)
따라서 Conic \(C^*_\infty\) 은 아래와 같이 표현할 수 있습니다.
\(C^*_\infty = \begin{pmatrix} 1 \\ i \\ 0 \end{pmatrix}\begin{pmatrix} 1 & -i & 0 \end{pmatrix} + \begin{pmatrix} 1 \\ -i \\ 0 \end{pmatrix}\begin{pmatrix} 1 & i & 0 \end{pmatrix} = \begin{bmatrix} 1 & 0 & 0 \\ 0 & 1 & 0 \\ 0 & 0 & 0 \end{bmatrix}\)
2.4.4 Angles on the projective plane#
사영 평면에서 \(C^*_\infty\)을 결정하면 다음과 같은 방법으로 유클리드 각을 측정할 수 있습니다.
위 수식은 사영 변환에서 불변량이며, \(l^TC^*_\infty m = 0\)이면 직선 \(l\)과 \(m\)은 직교합니다.
2.4.5 Recovery of metric properties from images#
원형점을 표준 위치 \((1, \pm i, 0)^T\)로 변환하면 사영 왜곡된 이미지에서 거리 속성을 복원할 수 있습니다. 그리고 Result 2.21.에 의해 실세상 평면과 복원된 이미지 사이의 변환이 닮음 변환임을 보일 수 있습니다.
Metric Rectification using \(C^*_\infty\)#
다음과 같이 \(C^*_\infty\)를 이용하여 사영 변환의 닮음 왜곡을 제외하고 사영과 아핀 구성 행렬을 모두 결정할 수 있습니다.
Metric rectification from affine distorted images#
이미지가 아핀 보정이 됐다고 가정하면, 거리 보정을 위해 원형점의 자유도 2를 결정하는 제약 조건 2개가 필요합니다. 실세상 평면에서 직각인 두 개의 이미지에서 제약 조건을 얻을 수 있습니다.
아핀 정정 이미지의 직선 \(l', m'\)이 실세계에서 직교하는 선 \(l, m\)에 대응한다고 가정하면 \(l'^TC^*_\infty m = 0, \mathbf{v} = \mathbf{0}\)을 이용할 수 있고, 바로 위에서 언급된 \(C^*_\infty\)’에 대한 공식을 다음과 같이 정리할 수 있습니다.
이것은 2 X 2 행렬 \(\mathbf{S} = KK^T\)에 대한 선형 제약조건이다. 행렬 \(\mathbf{S}\)는 대칭이므로 3개의 독립 원소를 가지며, scaling은 중요하지 않으므로 자유도는 2입니다.
따라서 두 선의 직교 조건은 \((l'_1m'_1, l'_1m'_2 + l'_2m'_1, l'_2m'_2)\mathbf{s} = 0\)으로 표기할 수 있습니다. 이러한 방식으로 두 개의 직교하는 선 쌍에서 제약조건 두 개를 얻으면, Cholesky Decomposition을 이용하여 \(K\)를 구해낼 수 있고, \(C^*_\infty\)’를 계산할 수 있습니다.
아래 해당 코드를 첨부합니다.
img = cv2.imread('./figures/chessboard.jpg', cv2.IMREAD_COLOR)
# select two orthogonal pairs
st = [np.array([1157, 676]), np.array([989, 638]), np.array([1055, 544]), np.array([1221, 582])]
ed = [np.array([989, 638]), np.array([1055, 544]), np.array([1221, 582]), np.array([1157, 676])]
st = np.append(st, np.ones((4,1), dtype=np.int32), axis=1)
ed = np.append(ed, np.ones((4,1), dtype=np.int32), axis=1)
l1 = np.cross(st[0], ed[0])
l2 = np.cross(st[1], ed[1])
m1 = np.cross(st[2], ed[2])
m2 = np.cross(st[3], ed[3])
# constraints
ortho_constraint = np.array([[l1[0]*m1[0], l1[0]*m1[1]+l1[1]*m1[0], l1[1]*m1[1]],
[l2[0]*m2[0], l2[0]*m2[1]+l2[1]*m2[0], l2[1]*m2[1]]
])
# decompose orthogonal constraint using SVD to gain S
U,S,V = np.linalg.svd(ortho_constraint, full_matrices=True)
S_matrix = np.diag(S)
# S = KK^T
# decompose S using Cholesky decomposition to gain K
K = np.linalg.cholesky(S_matrix)
K = K/K[1][1]
# Compute metric rectification matrix
H_m = np.eye(3)
H_m[:2,:2] = K
H_m = np.linalg.inv(H_m)
# recover the metric properties
dst = cv2.warpPerspective(img, H_m*H_A, (0,0))
cv2.line(img, st[0][:2], ed[0][:2], (255,0,0),3)
cv2.line(img, st[1][:2], ed[1][:2], (255,0,0),3)
cv2.line(img, st[2][:2], ed[2][:2], (0,0,255),3)
cv2.line(img, st[3][:2], ed[3][:2], (0,0,255),3)
concat = np.concatenate([img, dst], axis=1)
plt.imshow(concat)
<matplotlib.image.AxesImage at 0x169db0650>
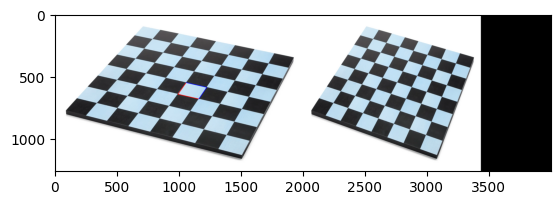
Angle measurement code#
위 보정된 이미지에서 좌표를 찍어 측정해본 결과입니다.
st = [np.array([992, 621]), np.array([872, 583])]
ed = [np.array([872, 583]), np.array([916, 492])]
st = np.append(st, np.ones((2,1), dtype=np.int32), axis=1)
ed = np.append(ed, np.ones((2,1), dtype=np.int32), axis=1)
conic = np.array([[1,0,0],
[0,1,0],
[0,0,0]])
l = np.cross(st[0], ed[0])
l = l/l[2]
m = np.cross(st[1], ed[1])
m = m/m[2]
# calulate the angle using conic
cos_theta = l.T@conic@m
val = np.sqrt((l.T@conic@l)*(m.T@conic@m))
cos_theta = cos_theta / val
theta = np.arccos(cos_theta) * 180 / np.pi
print('angle between l and m : ', theta)
cv2.line(dst, st[0][:2], ed[0][:2], (255,0,0),3)
cv2.line(dst, st[1][:2], ed[1][:2], (0,255,0),3)
plt.imshow(dst)
angle between l and m : 81.7667263783753
<matplotlib.image.AxesImage at 0x168e465d0>
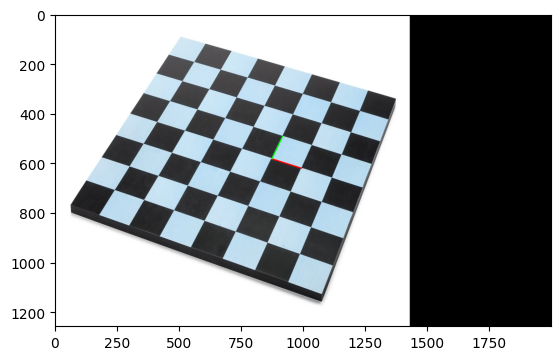
Metric rectification from original images#
아핀 왜곡된 이미지에서 거리 속성 복원한 위 사례와 유사하게, 원본 이미지에서 바로 거리 속성을 복원할 수 있습니다. 직선 \(l\)과 \(m\)을 실세상 평면에서 직교하는 직선의 이미지로 가정하면 \(l'^TC^*_\infty m = 0\)을 이용할 수 있고, 다음과 같이 \(C^*_\infty\)에 대한 선형 제약 조건을 얻습니다.
여기에서
\(\mathbf{c} = (a, b, c, d, e, f)^T\)는 6차원 벡터로 표기한 \(C^*_\infty\)의 conic matrix 입니다. 이러한 제약조건 5개로 5 X 6 행렬을 만들면 \(\mathbf{c}\)는 (\(C^*_\infty\)) null vector가 됩니다. 따라서 \(C^*_\infty\)는 실세계에서 직교하는 다섯 선 쌍에서 선형적으로 결정할 수 있습니다.
Stratification#
\(C^*_\infty\) 를 식별하여 원본 이미지에서 아핀 왜곡과 사영 왜곡을 한 번에 정정하는 방법을 배웠습니다. 그리고 사영 왜곡을 먼저 제거하고 아핀 왜곡을 제거하는 방법도 배웠습니다. 이러한 2단계 접근 방식을 Stratification 이라고 합니다. 비슷한 접근 방식을 3차원에서도 적용할 수 있습니다.
Reference#
Multiple view geometry in computer vision chapter 2.7