4. Data Flow#
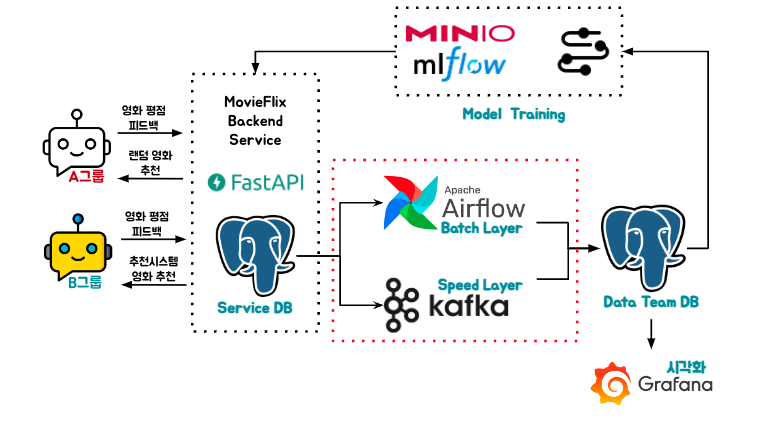
서비스 모니터링 및 Model Training을 위해 Data Team DB로 데이터를 옮기는 과정입니다.
Airflow#
람다 아키텍처에서 Batch Layer를 담당합니다.
1시간마다 한번 테이블에서 kafka를 통해 생성된 데이터를 Delete 하고 Service DB에서 데이터를 읽어 Insert합니다.
kafka를 통해 생성된 데이터의 중복, 유실을 보정하기 위한 장치입니다.
Dag Repository를 통해 Airflow Dag을 관리합니다.
git-sync
를 통해 Repository에 반영된 코드만 Airflow에 반영될 수 있도록 합니다.
Dynamic Dag Generation#
# 경로의 json파일을 읽어 해당 내용에 따라 Dag를 생성합니다.
for filename in os.listdir("/opt/airflow/dags/etl-dags"):
if filename.endswith(".json"):
dag_id = filename[:-5]
with open(f"/opt/airflow/dags/etl-dags/{filename}", "r") as f:
config = json.load(f)
table_name = config["table_name"]
schedule_interval = config["schedule_interval"]
catchup = config.get("catchup", "False") == "True"
start_date_str = config["start_date"]
start_date = datetime.strptime(start_date_str, "%Y-%m-%d")
globals()[dag_id] = DAG(
dag_id,
default_args=default_args,
description=f"Load data into {table_name}",
schedule_interval=schedule_interval,
start_date=start_date,
catchup=catchup,
is_paused_upon_creation=False,
)
load_data_task = PythonOperator(
task_id=f"load_data_into_{table_name}",
python_callable=run_transfer,
op_kwargs={"table_name": table_name},
dag=globals()[dag_id],
)
정형화된 로직에 따라 여러 Dag를 생성해야 할 경우 유용할 수 있습니다.
Kafka#
람다 아키텍처에서 Batch Layer를 담당합니다.
Airflow로 구현하기에 적절하지 않은 실시간 데이터 생성을 담당합니다.
Source Connector를 사용하여 Source DB의 데이터를 브로커의 토픽으로 publish 합니다.
Sink Connector를 사용하여 토픽의 데이터를 Subscribe하여 Target DB에 전달합니다.
topic 구성 예시
postgres-source-feedbacks
Source
"table.whitelist": "feedbacks",
"topic.prefix": "postgres-source-",
Sink
"topics": "postgres-source-feedbacks",
/MovieRecommend/project/kafka/connector_preprocess
경로의 json파일을 구성해야합니다.